Table of Contents
AI Agents in Enterprise: From Investment to Impact
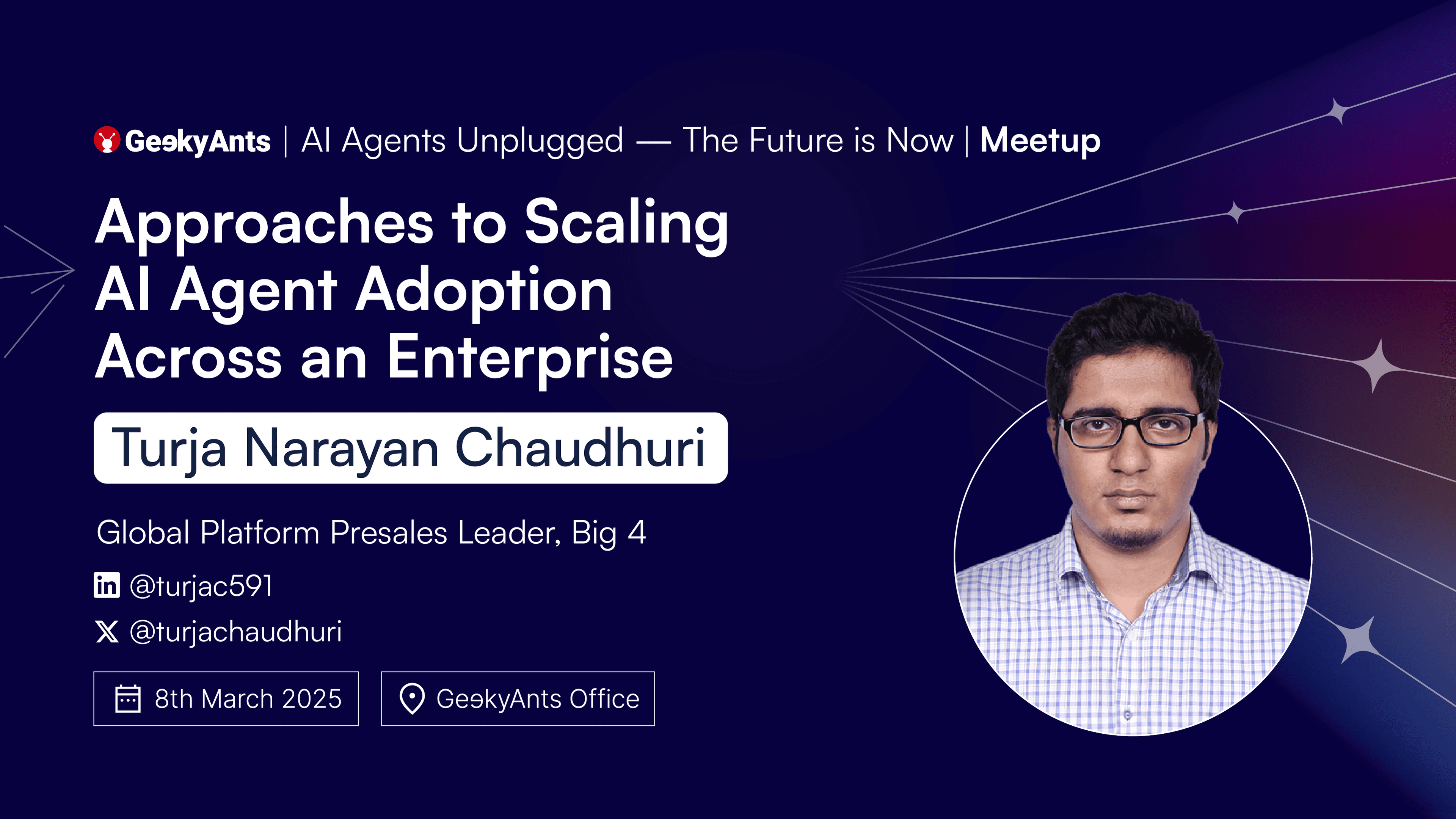
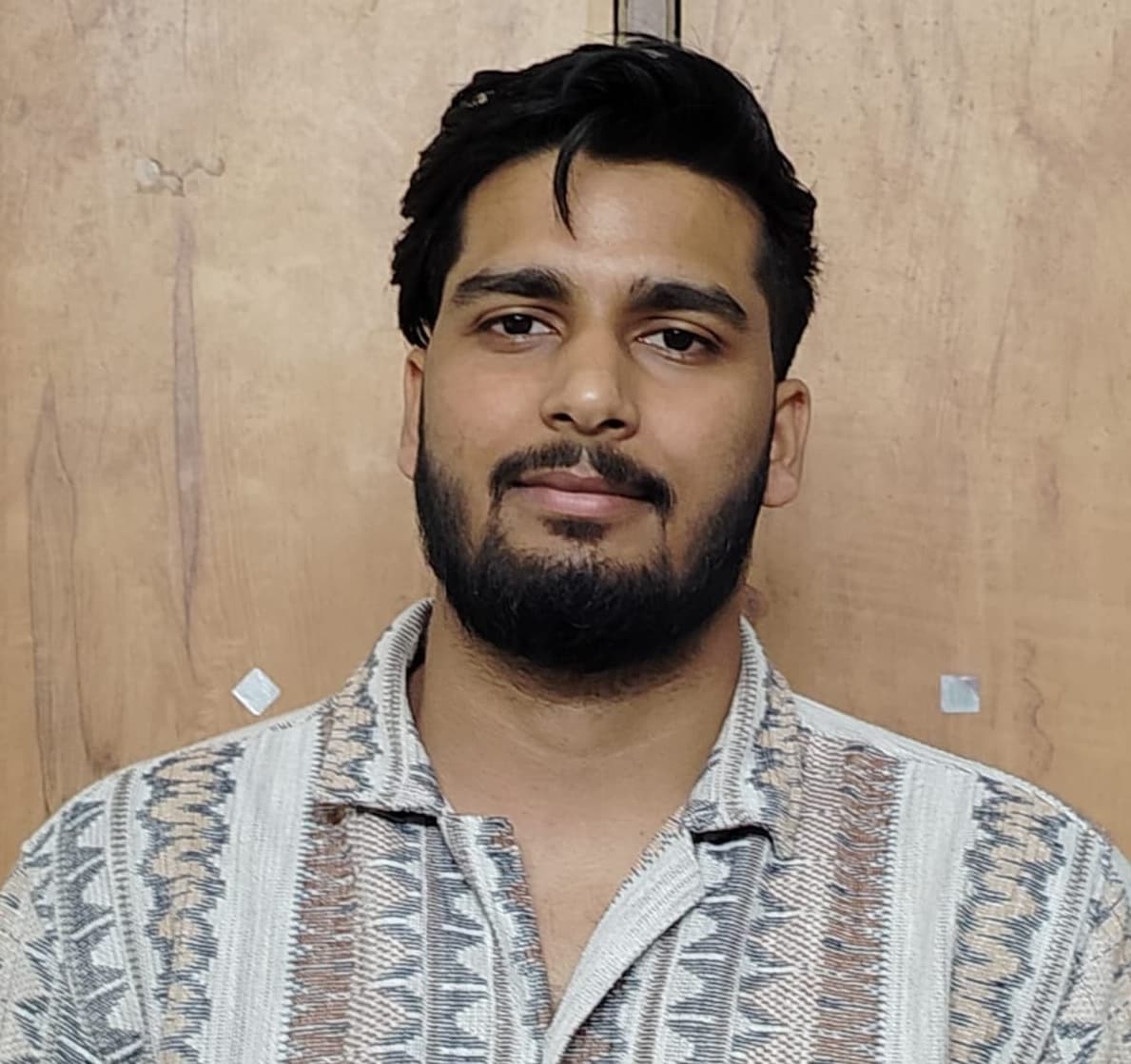
Book a call
Editor’s Note: This blog is an adapted narrative based on insights shared by Turja Narayan Chaudhuri during an internal strategy session at GeekyAnts. While refined for clarity, it reflects his deep dive into how AI agents and platforms are transforming enterprise adoption and impact.
AI funding has reached unprecedented levels, with billions flowing into companies like OpenAI, X.AI, and SafeGraph. OpenAI alone saw its valuation soar from $1.6 billion in 2023 to $3.7 billion in 2024, doubling its revenue in a year. While startups and investors capitalize on this momentum, enterprises remain cautious. A PwC survey of 1,500 CEOs revealed that 66% have not seen a tangible return on their Gen AI investments. Despite the promise of AI-driven transformation, many organizations struggle to translate innovation into measurable business value, facing scalability, integration, and governance challenges.
The issue is not AI’s potential—it is how enterprises deploy, manage, and monetize it effectively. AI agents provide a fresh approach, shifting from static models to autonomous, context-aware systems. These systems can make decisions and act in real time. By integrating AI agents into business environments, companies can connect AI’s potential with real results. This change isn’t just about using AI for innovation; it is about using it to develop scalable, efficient, and revenue-generating solutions.
The AI Investment Surge: Why Enterprises Are Struggling
The AI industry is growing faster than SaaS, with AI-driven companies reaching revenue milestones one-third of the time compared to traditional SaaS businesses. Yet, despite this rapid growth, enterprises face significant barriers to adoption:
- High investment, low return: Only 34% of CEOs report positive ROI from AI initiatives.
- Enterprise complexity: Unlike consumer AI tools, enterprise AI must integrate with legacy systems, ensure security, and deliver consistent results.
- The AI dilemma: Companies risk falling behind competitors if they delay adoption, yet early investments without clear implementation strategies lead to inefficiencies.
The key challenge is not AI itself—it’s how enterprises deploy and scale AI to deliver real-world business value.
AI Agents as the Missing Link for Enterprise AI Adoption
What Are AI Agents?
AI agents are autonomous decision-making systems designed to go beyond static AI models. Unlike traditional AI, which processes inputs and delivers predefined outputs, AI agents analyze context, plan, and execute tasks in real time.
These agents bring three critical capabilities to enterprise AI:
- Context retention – AI agents learn and adapt rather than restarting with every interaction.
- Tool execution – They can integrate with APIs, databases, and enterprise systems to perform real tasks.
- Autonomous decision-making – AI agents not only provide insights but take action based on business rules.
By addressing the shortcomings of traditional AI models, AI agents help enterprises unlock AI’s full potential while maintaining efficiency and control.
The Role of Platforms in Scaling AI Agents
Enterprise AI adoption is not just about deploying AI models—it’s about creating scalable AI infrastructure.
Why Enterprises Need AI Platforms:
- Reduces developer workload: Internal AI platforms centralize key services, so teams don’t need to rebuild AI solutions repeatedly.
- Ensures governance and compliance: AI adoption at scale requires security, standardized APIs, and regulatory compliance measures.
- Optimizes cost and efficiency: AI platforms allow teams to share AI models and reuse pre-built solutions, reducing duplication and inefficiencies.
Without a structured platform, AI adoption remains fragmented and difficult to scale—leading to the very ROI challenges many CEOs report.
The Three Stages of AI Agent Implementation
Enterprise AI adoption is not an instant transformation—it follows a structured path that moves from visibility to scalability to profitability. Successful implementation requires a clear strategy to integrate AI agents efficiently, maximize their utility, and ensure long-term financial viability.
AI Agent Index – Centralized AI Knowledge Base
Many enterprises invest in AI but lack a systematic way to track and utilize existing models across teams. This results in fragmented AI efforts, redundant projects, and inefficiencies. The MIT AI Agent Index addresses this challenge by mapping deployed AI agents, preventing duplication, and improving reusability across organizations. Enterprises can apply this approach internally by creating a centralized AI index, allowing teams to discover, share, and build upon existing AI models, streamlining development and reducing time-to-market for new AI solutions.
AI Agent Marketplace – Enabling Reuse and Collaboration
An AI marketplace elevates adoption by making AI agents discoverable, reusable, and scalable across teams. Instead of siloed AI applications, organizations can create a structured marketplace where teams can access pre-built AI models, automate workflows, and integrate solutions seamlessly. Companies like HubSpot’s Agent AI Network showcase how enterprises can facilitate AI deployment while maintaining governance. However, marketplaces require strict security, compliance, and access controls to ensure that AI agents align with enterprise-grade standards.
AI Agent Monetization – Turning AI into a Profit Center
AI incurs ongoing costs, making a clear monetization strategy essential. Enterprises can adopt three primary pricing models to sustain AI operations:
- Flat Subscription – A fixed licensing fee, mirroring traditional SaaS.
- Consumption-Based – Charges tied to usage metrics, such as token-based billing.
- Outcome-Based – Payment only when AI achieves a measurable business goal.
Selecting the right model ensures AI accessibility while maintaining cost efficiency and profitability. As AI agents mature, organizations must balance accessibility with financial sustainability, ensuring that AI investments translate into long-term business value.
AI Agent Management: Scaling AI at an Enterprise Level
Deploying AI agents is only the first step—managing them at scale is the real challenge. As enterprises expand AI adoption, they must ensure that agents are monitored, optimized, and governed effectively. Without structured management, AI deployments risk becoming fragmented, inefficient, and difficult to control.
The AI Agent Factory Concept
An AI Agent Factory provides a standardized approach to creating, deploying, and maintaining AI agents at scale. It addresses key enterprise challenges by:
- Tracking AI performance – Continuously monitoring AI outputs to ensure reliability and accuracy.
- Debugging failures – Identifying workflow issues and providing rapid intervention strategies.
- AI governance – Enforcing compliance, security policies, and risk management protocols.
By implementing an AI Agent Factory, enterprises gain full visibility into their AI ecosystem, reducing operational risks while improving AI-driven efficiency.
The Role of the AI Agent Management Platform (AMP)
Managing AI agents requires more than just oversight—it demands a centralized control system. An AI Agent Management Platform (AMP) enables enterprises to:
- Monitor large-scale AI deployments with real-time insights.
- Manage AI updates and iterations efficiently without disrupting workflows.
- Ensure compliance with enterprise policies, reducing regulatory and security risks.
A structured AI lifecycle management strategy is essential for enterprises looking to scale AI effectively. Without it, AI initiatives become disorganized, expensive, and difficult to sustain, limiting their long-term impact.
The Future of Enterprise AI: What Comes Next?
AI agents are shifting from experimental tools to core enterprise assets, with 2025 set to be a defining year for large-scale adoption. As AI infrastructure matures, costs are dropping significantly, making enterprise-wide deployment more viable than ever. The rise of Agentic Enterprises will see AI agents handling decision-making, automation, and execution, replacing inefficient manual workflows. However, AI will not eliminate human oversight—it will augment enterprise intelligence, allowing companies to scale faster while maintaining control. Businesses that master AI agent deployment, governance, and monetization will set the standard for the next phase of digital transformation.
Conclusion
AI agents have moved beyond proof-of-concept experiments and are now reshaping enterprise AI strategies. As organizations transition from isolated deployments to scalable AI ecosystems, structured implementation through AI indexing, marketplaces, and management platforms will determine long-term success. The rise of Agentic Enterprises will redefine workflows, enabling AI to drive decision-making, automation, and efficiency at scale. However, the real differentiator will be how well enterprises integrate AI with governance, monetization, and operational control to maximize impact.
Looking to integrate AI agents into your enterprise? Partner with GeekyAnts to build scalable, AI-driven business solutions.
Dive deep into our research and insights. In our articles and blogs, we explore topics on design, how it relates to development, and impact of various trends to businesses.