Table of Contents
Evolution of Code Reviews: From Manual Checks to AI Collaboration
Author
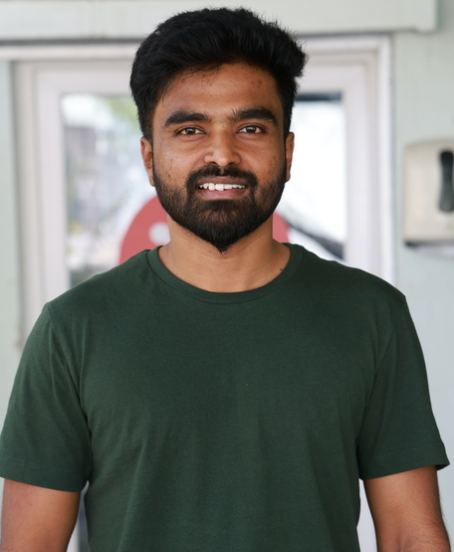
Date
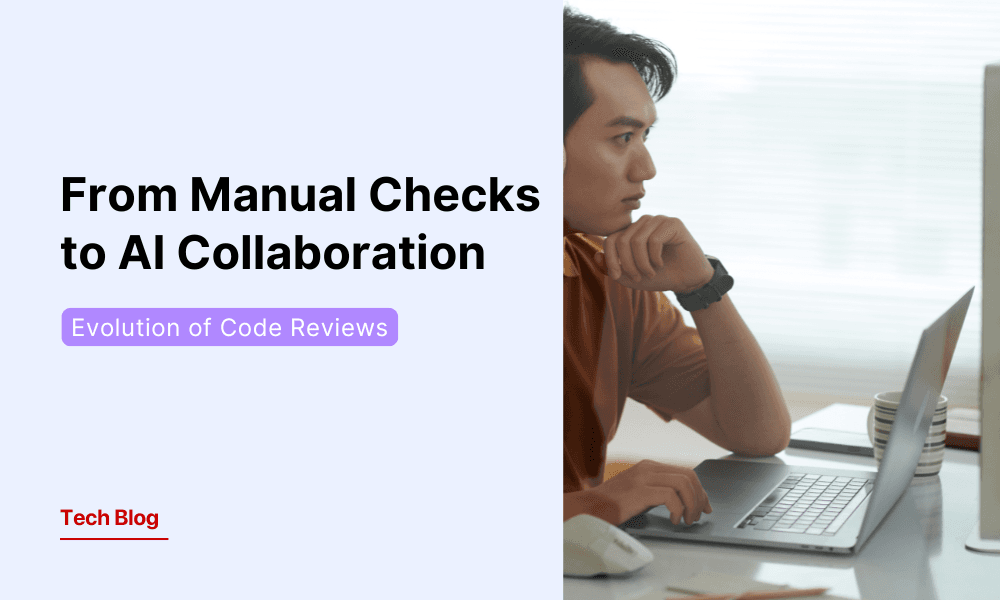
Book a call
Code reviews have always been a cornerstone of quality software development. This critical process—where developers examine each other's code for errors, improvements, and adherence to standards—has undergone a remarkable transformation. What began as manual, often laborious, peer reviews has evolved into sophisticated, AI-assisted workflows that are reshaping how development teams collaborate and ensure code quality.
Today, we find ourselves at a fascinating inflection point. Tools like GitHub Copilot Review and CodeRabbit are moving into the mainstream, marking the third major shift in code review practices: from entirely manual reviews, through rule-based automation, and now into the era of AI-driven assistance. Let's explore this journey and consider what it means for the future of building great software.
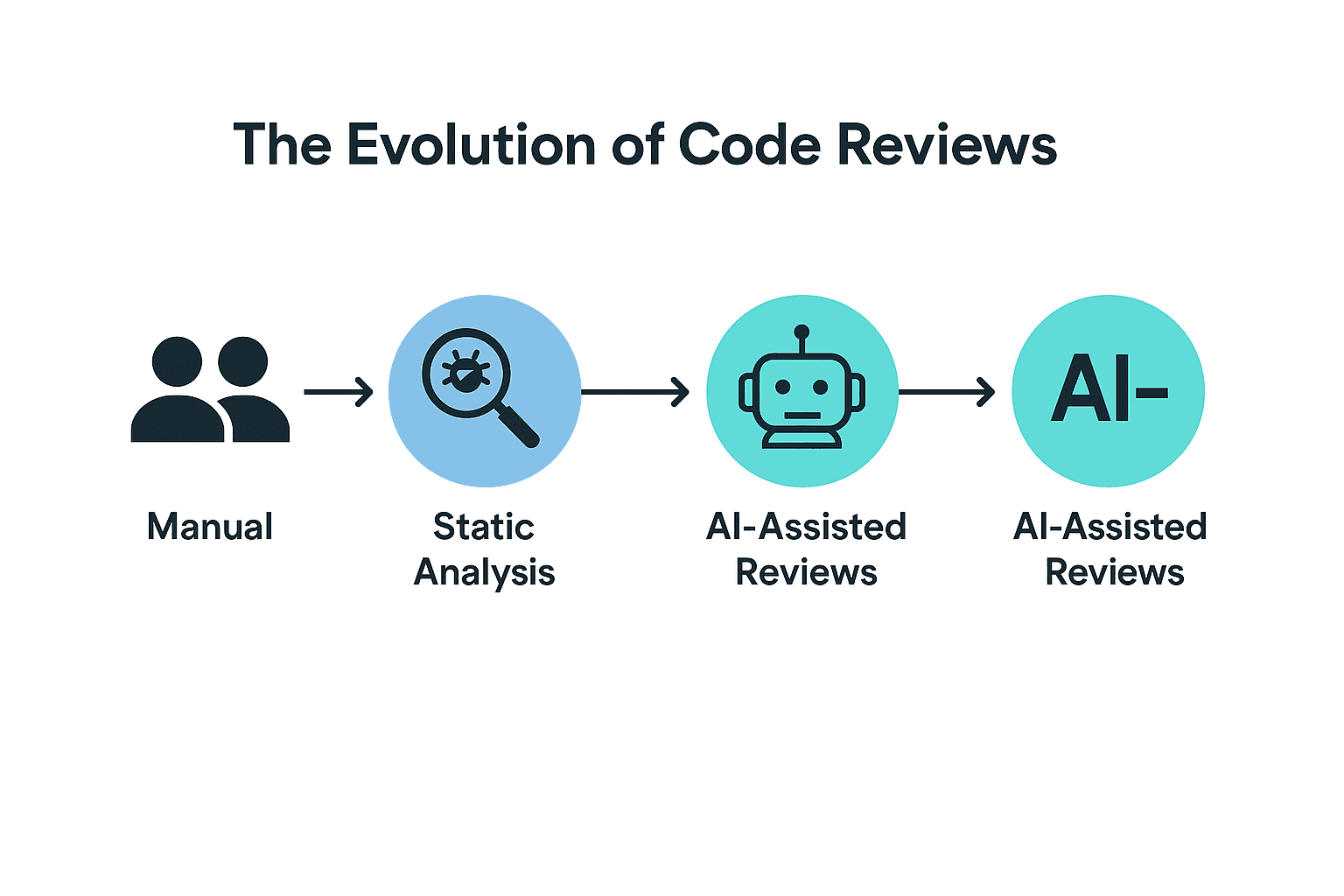
The Early Days: Manual Code Reviews
Origins of Peer Review in Software
The roots of formal code review trace back to the 1970s at IBM. These early "inspections" often involved developers gathering in a room, poring over physical printouts line by line. Imagine developers huddled around a table covered in code listings – a thorough, personal, but incredibly time-consuming process, especially for complex systems.
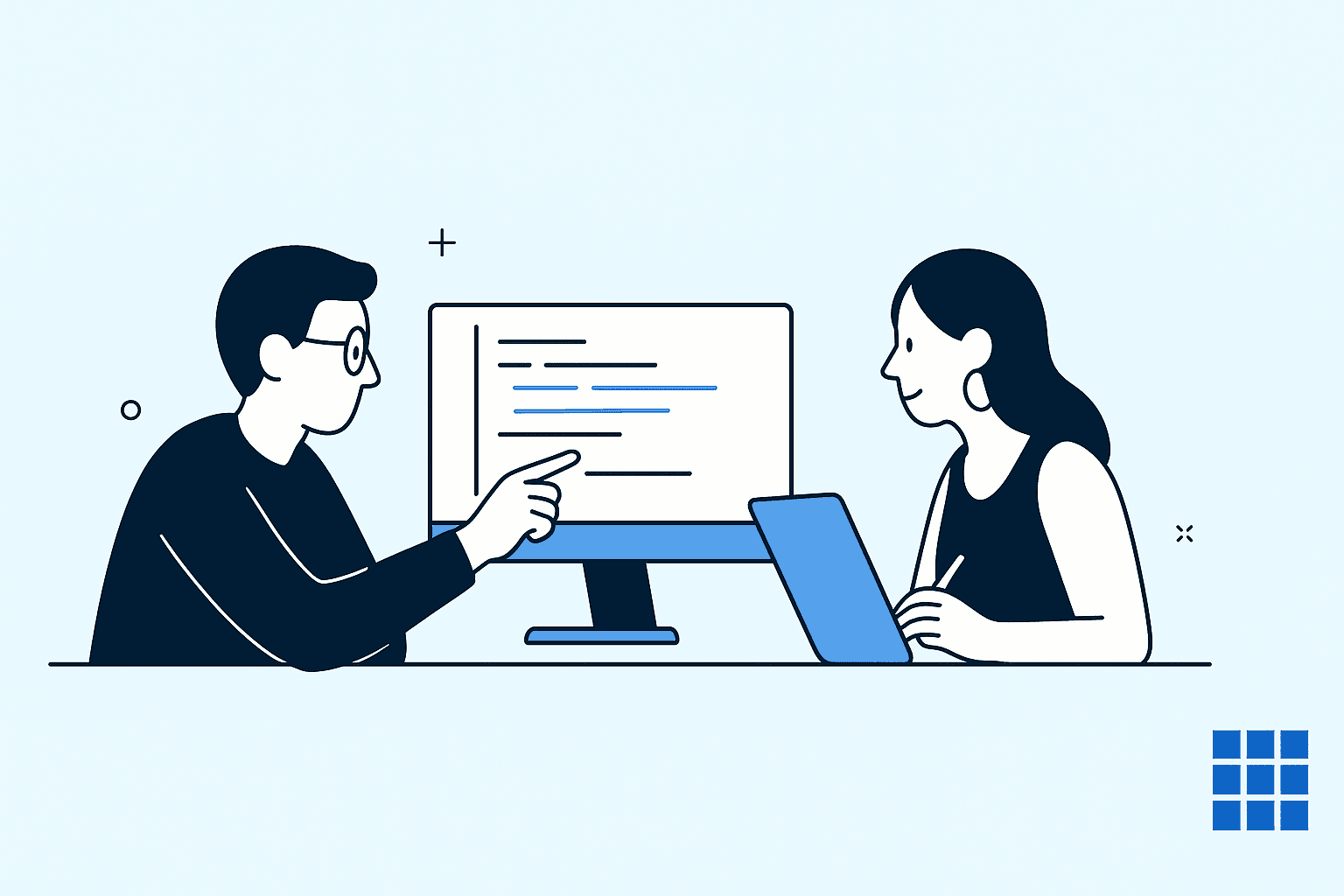
As practices matured, several manual approaches became common:
- Over-the-shoulder reviews: A developer walks a colleague through their code, explaining the logic. Quick and informal, offering immediate feedback but often missing deeper issues.
- Formal code walkthroughs: Structured meetings where the author guides multiple reviewers through the code. More thorough, but also very time-intensive.
- Pair programming: Popularized by Extreme Programming, two developers work on the same code simultaneously, providing continuous, real-time review.
The Challenges of Going Manual
Despite their value in catching bugs and sharing knowledge, manual reviews presented significant hurdles:
- Time Sink: Reviews could easily consume 20-30% of a developer's time, creating bottlenecks.
- Subjectivity: Feedback quality varied wildly based on the reviewer's expertise, mood, or even personal coding style preferences.
- Inconsistency: Different reviewers might focus on entirely different aspects – one on formatting, another on logic.
- Reviewer Fatigue: Maintaining focus during long review sessions is hard, leading to diminishing returns.
- Knowledge Silos: Effective review often depended on the availability of specific team members with the right expertise.
As software complexity grew, these limitations became unsustainable, pushing the industry toward automation.
Automating the Basics: The Rise of Linters and Static Analysis
The early 2000s brought the first wave of automation. Static analysis tools and linters – programs analyzing source code without running it – emerged to handle the more repetitive, rule-based aspects of code review.
Key Tools That Changed the Game
Familiar names began automating checks across languages:
- ESLint/JSLint: Enforced JavaScript style rules and caught common errors.
- Pylint: Did the same for Python codebases.
- Checkstyle: Helped Java developers maintain consistent standards.
- PMD/FindBugs: Identified common Java programming flaws.
- SonarQube: Went beyond linting to offer deeper code quality metrics and security vulnerability detection across multiple languages.
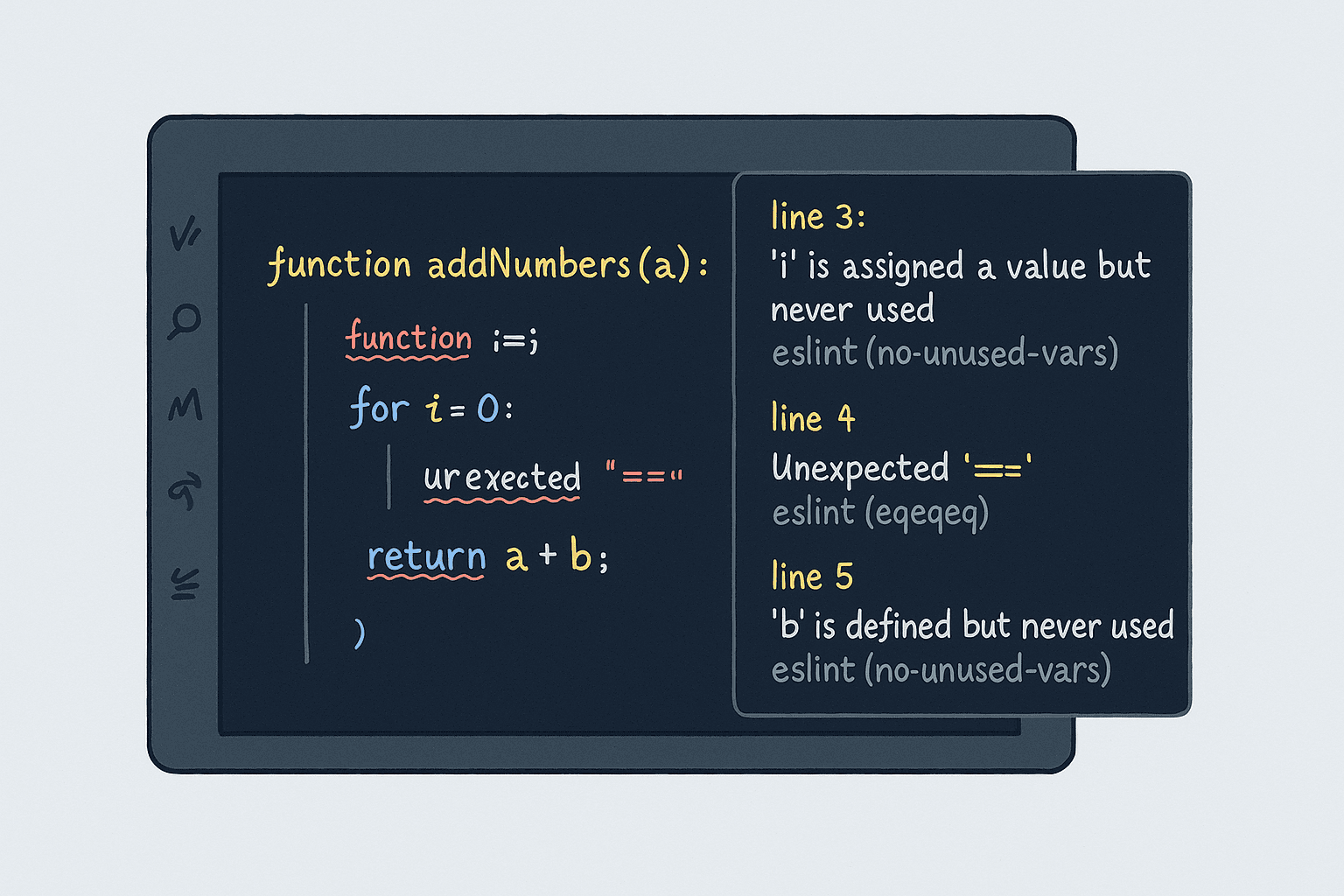
Integration: The Real Power Unleashed
These tools became truly powerful when integrated into Continuous Integration/Continuous Deployment (CI/CD) pipelines and version control systems (like Git via GitHub, GitLab, Bitbucket). This allowed teams to:
- Automatically enforce quality gates within the development workflow.
- Ensure consistent styling and documentation.
- Catch potential bugs and security issues early.
- Track code quality metrics over time.
Webhooks and APIs connected these tools directly to pull/merge requests, triggering reviews automatically and delivering feedback right where developers work. No more context switching to check separate dashboards!
Handling Multi-Language Projects
As projects increasingly used diverse tech stacks (e.g., JavaScript frontends, Python backends, Swift mobile apps), static analysis tools evolved to support multiple languages, often within a single platform. While powerful, configuring language-specific rule sets still required significant team effort.
Benefits and Lingering Limitations
Automation brought clear advantages:
- Consistency: Reliably caught syntax errors, style issues, and anti-patterns.
- Objectivity: Reduced debates over subjective formatting preferences.
- Efficiency: Freed up human reviewers from mundane checks.
- Scalability: Handled growing codebases easily.
- Speed: Provided near real-time feedback.
However, static analysis had its limits:
- It struggled with nuance requiring business context or logical understanding.
- It generated false positives needing manual verification.
- It couldn't identify logical flaws, architectural weaknesses, or poor algorithmic choices.
- It lacked insight into developer intent.
- It relied heavily on predefined rules, missing novel issues.
Experts estimated these tools addressed only about 30% of what a comprehensive review should catch. The rest required human judgment – until AI entered the scene.
The Current Shift: AI-Powered Code Reviews
The 2020s ushered in a new era with AI-powered tools capable of providing more intelligent, context-aware feedback that goes far beyond simple rule-checking.
Pioneering AI Code Review Tools
Several tools are leading this revolution:
- GitHub Copilot Review: Tightly integrated into the GitHub ecosystem, Copilot Review (part of the GitHub Copilot subscription) uses large language models (LLMs) to analyze pull requests. It comments directly on code changes, suggesting fixes for bugs, security vulnerabilities, and quality issues across many languages. Its seamless integration makes it a natural fit for teams on GitHub.
- CodeRabbit: Another powerful AI tool working with both GitHub and GitLab. CodeRabbit focuses on intelligent inline suggestions, team collaboration features, and extensive customization. It uses LLMs to understand complex code context and can even offer auto-fixes for certain issues.
- Other notable players include: CodiumAI (focusing on test generation and coverage), Amazon CodeWhisperer (strong on security and AWS best practices).
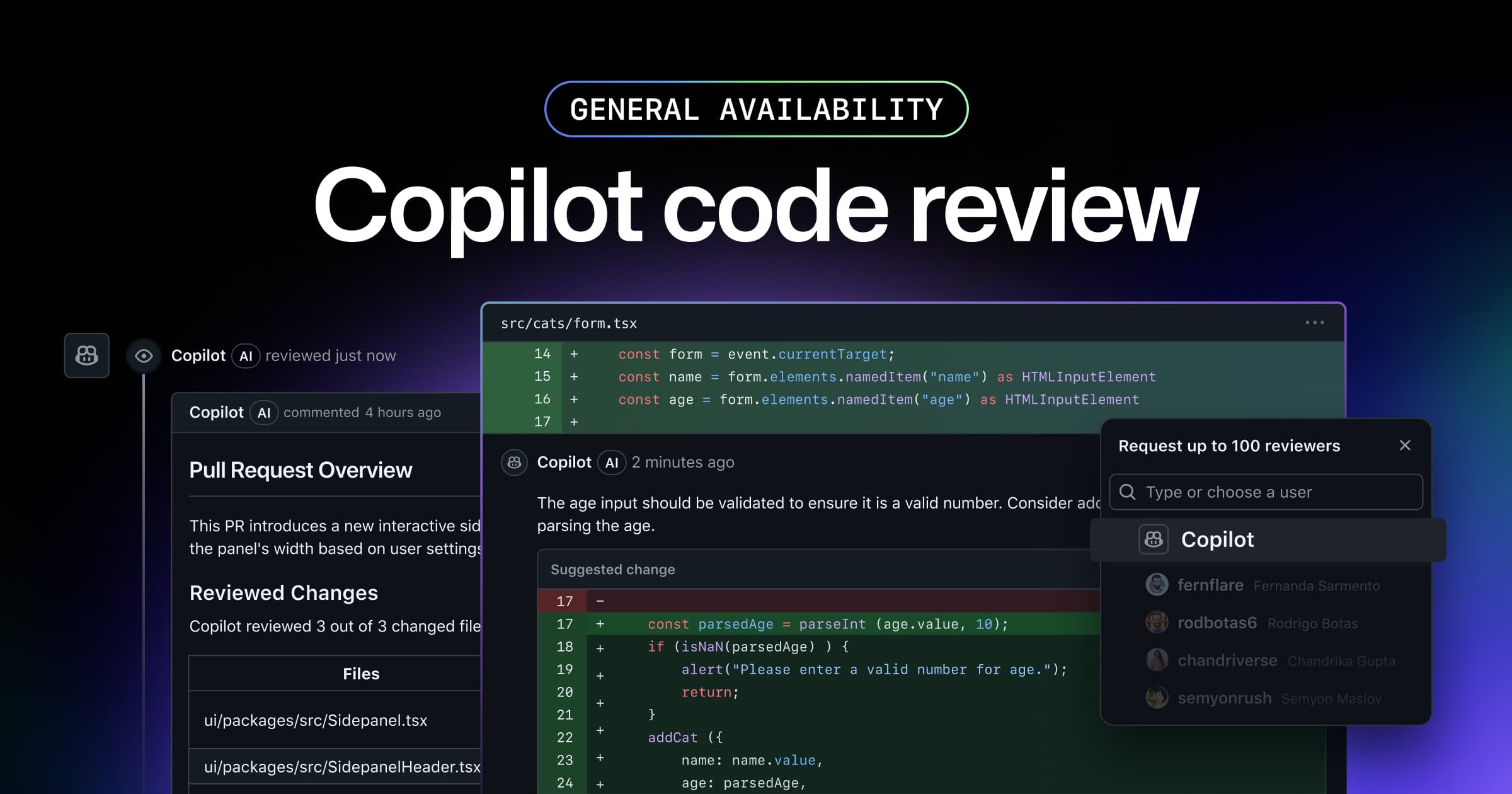
How AI is Transforming Reviews
What makes AI different from traditional static analysis?
- Contextual Understanding: AI can grasp the broader context of changes, not just isolated lines.
- Learning Capabilities: These tools learn from the codebase, accepted changes, and team preferences over time.
- Natural Language Processing (NLP): AI can interpret comments, documentation, and commit messages to better understand developer intent.
- Predictive Analysis: Some tools can anticipate potential future problems arising from current code patterns.
A Closer Look: Copilot Review vs. CodeRabbit
- GitHub Copilot Review Workflow: A developer opens a PR, clicks "Generate review," and Copilot adds comments with suggestions and explanations directly to the PR for discussion and resolution. Simple and integrated.
- CodeRabbit Workflow: Integrates via a GitHub App or GitLab connection, automatically reviewing PRs/MRs upon creation or update. It offers deep customization of review rules and collaboration features, along with auto-fix options.
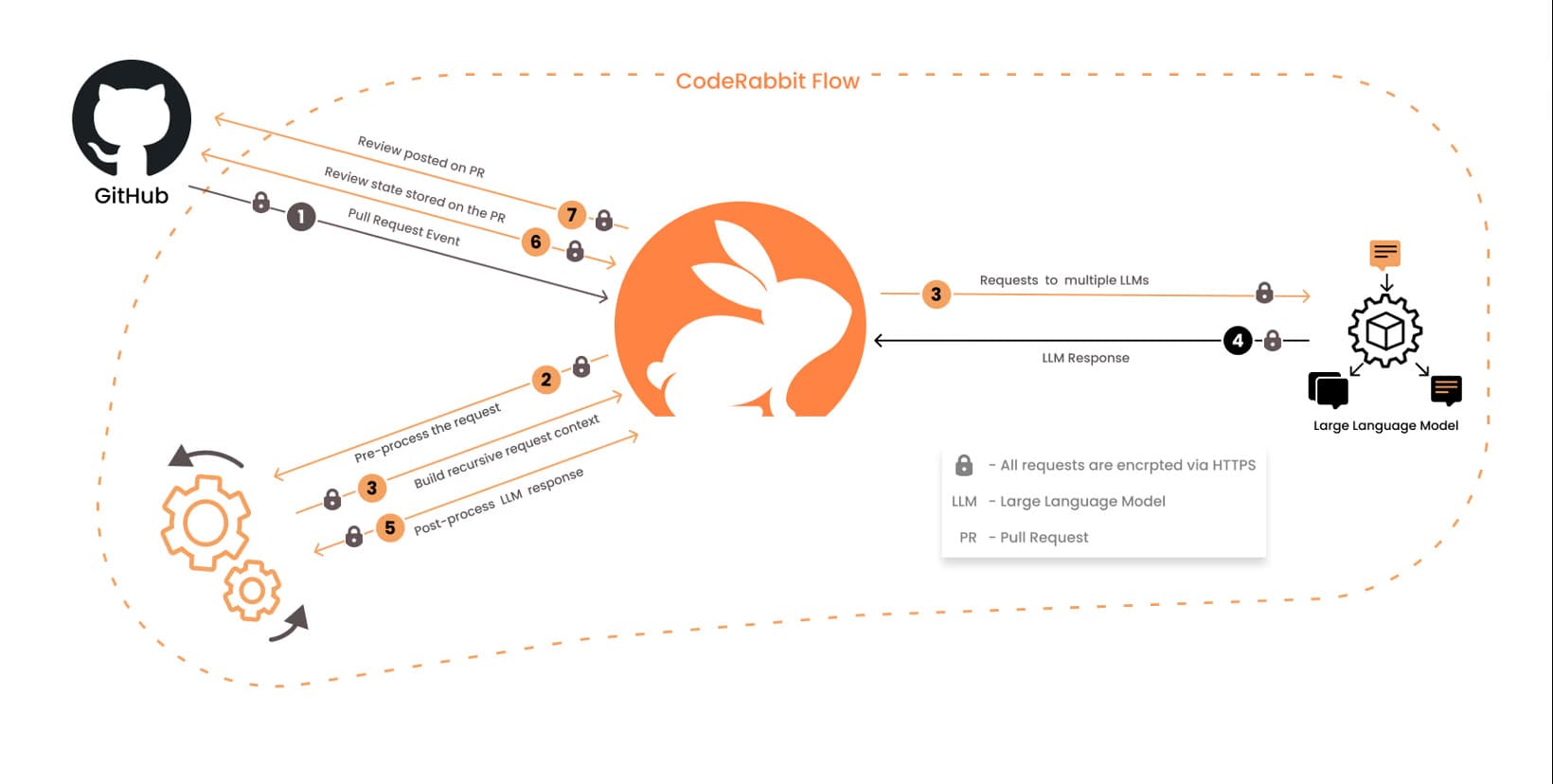
Real-World Impact
The benefits are becoming clear. Early reports around tools like GitHub Copilot Review suggested teams could:
- Resolve issues significantly faster (e.g., 15% faster).
- Merge pull requests more quickly (e.g., 33% faster).
- Identify substantially more edge cases and potential bugs.
Similarly, teams using tools like CodeRabbit often report:
- Reductions in time spent on reviews (e.g., up to 25%).
- Improved detection of security vulnerabilities.
- More consistent code quality, especially in larger teams.
By automating identification of common issues, AI allows human reviewers to focus their valuable time on complex logic, architecture, and alignment with business requirements.
Challenges and Limitations of AI Assistance
Despite the impressive progress, AI code review tools aren't a silver bullet. Important challenges remain:
Technical Limitations
- Context Boundaries: AI often struggles with system-wide implications or complex interactions between microservices.
- Domain Knowledge: AI typically lacks deep understanding of specific business domains or niche industry regulations unless specifically trained.
- Novelty Aversion: AI might favor conventional solutions seen in training data, potentially discouraging innovative approaches.
- False Confidence: AI can present incorrect suggestions assertively, potentially misleading less experienced developers.
Language and Framework Diversity
- Keeping Pace: The rapid evolution of languages and frameworks means AI models constantly need updating.
- Niche Technologies: Domain-specific languages or highly specialized frameworks might not be well-understood by general AI models.
- Multi-Language Complexity: While improving, AI can struggle with intricate interactions at the boundaries between different languages within a single project.
- How Tools Adapt: Both Copilot Review (leveraging broad training data) and CodeRabbit (offering language-specific custom rules) attempt to address this, showcasing different strategies for managing diversity.
Practical Concerns
- Security & Privacy: Sending proprietary code to third-party AI services requires careful consideration of data security and IP protection.
- Overreliance: Teams might become overly dependent on AI, potentially weakening developers' own critical review skills over time.
- Integration & Cost: Implementing these tools requires technical effort, potential workflow changes, and often involves subscription costs.
Ethical Considerations
- Bias: AI models can inherit and amplify biases present in their training data (e.g., favoring certain coding styles).
- Attribution: Who gets credit when AI suggests significant improvements?
- Skill Development: How do junior developers learn the nuances of review if AI handles the basics?
- Team Dynamics: Introducing an "AI reviewer" can alter collaboration, knowledge sharing, and mentorship patterns.
These challenges emphasize that AI is currently best viewed as a powerful assistant, augmenting rather than replacing human expertise.
The Future: Synergistic AI + Human Collaboration
The most effective path forward lies in optimizing the collaboration between AI and human reviewers. How can we achieve this synergy?
Effective Collaboration Models
Leading teams are adopting hybrid approaches:
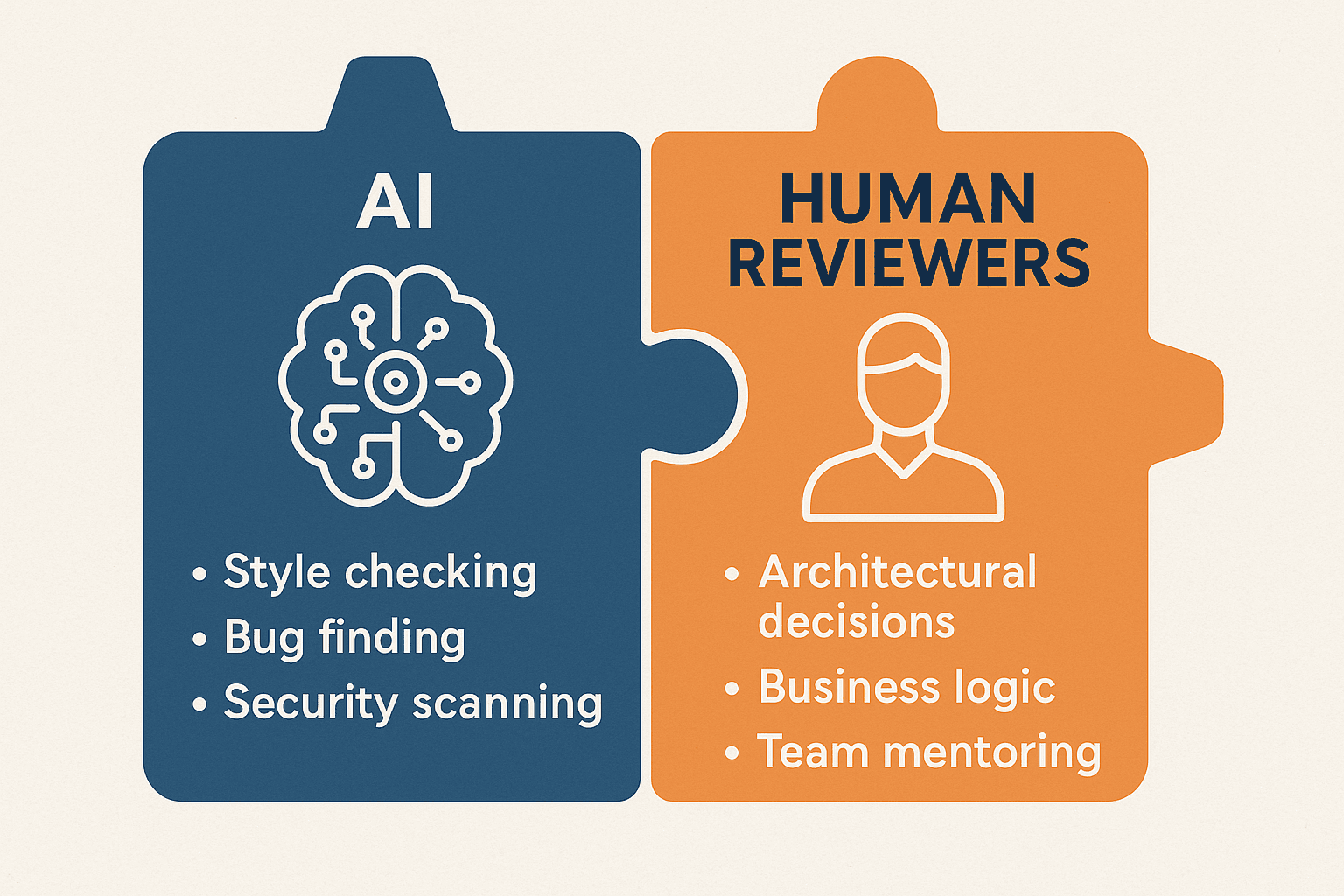
- AI Handles the Baseline: Let AI manage style consistency, common bugs, potential security flaws, and simple performance checks.
- Humans Focus on Strategy: Human reviewers concentrate on architecture, business logic correctness, long-term maintainability, usability, and complex edge cases – areas requiring deep understanding and critical thinking.
- Feedback Loops: Humans validate or correct AI suggestions, helping the AI improve while refining their own understanding.
- Customization: Tailor AI tools to understand team-specific standards, patterns, and business context.
GitHub's vision for Copilot Review exemplifies this: AI provides the first pass, human reviewers validate AI feedback and add higher-level insights, shifting focus from syntax to strategy.
What's Next on the Horizon?
- Predictive Analysis: AI identifying potential future issues based on current trends.
- Personalized Feedback: AI tailoring suggestions to a developer's experience level.
- Natural Language Interaction: Asking questions about code in plain English (e.g., GitHub Copilot Chat).
- AI-Guided Refactoring: Tools actively helping restructure code based on reviews.
- Deeper Lifecycle Integration: Connecting review insights to project planning and technical debt management.
Conclusion: Embracing Smarter Collaboration
The evolution of code review – from manual inspections to AI-powered collaboration – represents a profound shift in software development. Each phase tackled specific challenges: manual reviews established the why (quality, collaboration), static analysis improved the consistency and efficiency, and AI now brings deeper understanding and context.
The future isn't about choosing between humans or AI; it's about leveraging both intelligently. AI can handle the repetitive and pattern-based checks with superhuman speed and consistency, freeing developers to apply their creativity, critical thinking, and domain expertise to the challenges that truly require human intelligence.
Organizations that effectively integrate AI assistance into their code review process stand to gain a significant competitive advantage through faster delivery, higher quality, and more innovative products. The key is thoughtful adoption – viewing AI not just as a tool, but as a collaborative partner in the quest to build better software.
How is your team adapting to this new era of code review?
Dive deep into our research and insights. In our articles and blogs, we explore topics on design, how it relates to development, and impact of various trends to businesses.