Analysing AI App Development Dynamics in the UK: Local vs. Global
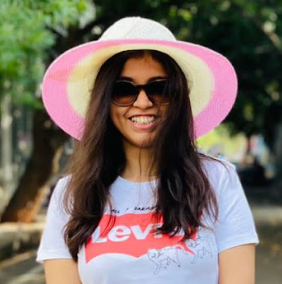
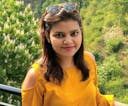
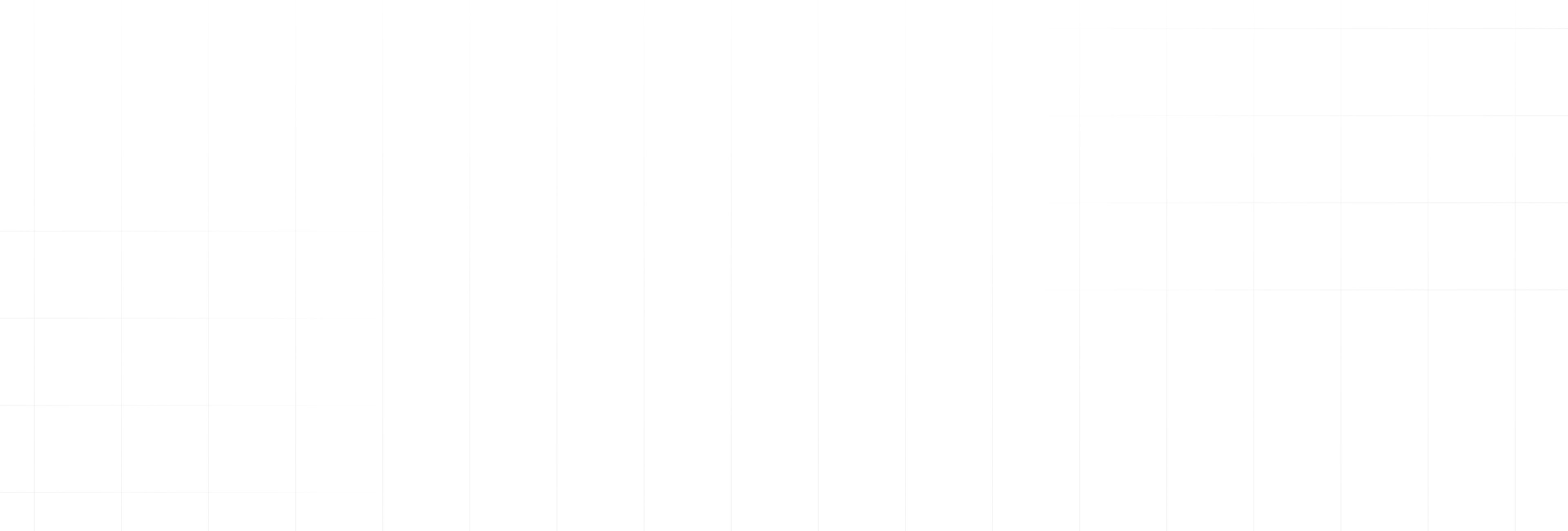
The UK’s AI Safety Summit of 2023 presented the idea of AI Globalism, an era where AI is accessible to everyone. Rishi Sunak also underlined the desire to establish the UK’s position as a leading AI juggernaut. His focus was on innovation and safety. However, he did mention that legislation cannot be expected soon. Clarity was needed on how AI models will be used and regulated in the UK.
In the midst of this all, considering the unique dynamics of local populations, AI Localism is also gaining traction. For companies and businesses planning to use AI-powered models in their websites or mobile applications, finding a middle ground between these two seemingly polar opposite ideas is crucial. That is what we will discuss in this blog.
We will also discuss strategies on when to opt for the global or local lens, expected regulations that can influence decisions related to the usage of AI in the UK, and current usage of AI by UK companies.
Globalism vs Localism of AI in the UK
AI globalism promotes a collaborative, boundary-spanning approach to AI development. Localism of AI advocates the importance of adapting AI technologies to suit the unique characteristics and requirements of individual regions or communities.
Here is a comparison table explaining the differences.
Aspect | AI Globalism | AI Localism |
---|---|---|
Collaboration | Emphasises global collaboration and knowledge sharing. | Prioritises tailoring AI solutions to local needs and contexts. |
Standards | Advocates for universal standards and ethical guidelines. | Values, cultural sensitivity, and local ethical considerations. |
Problem-Solving | Focuses on addressing global challenges with AI solutions. | Aims to solve local challenges specific to individual regions. |
Market Accessibility | Promotes global accessibility of AI innovations. | Prioritises the control of data and addresses local economic needs. |
Cultural Nuances | Gives importance to a global perspective, potentially overlooking local cultural nuances. | Recognises and respects local cultural values and diversity. |
Data Sovereignty | Less emphasis on data sovereignty; prioritises global data sharing. | Prioritises local control and ownership of data. |
Community Engagement | Emphasises collaboration globally. | Advocates for involving local communities in AI development. |
Challenges Addressed | Targets global challenges such as climate change and healthcare disparities. | Tailors solutions to address specific challenges in local industries and societies. |
Illustrating the application of AI Localism, DeepMind has successfully employed this approach in various products and services. Notably, the DeepMind Health team crafted AI systems aiding doctors in disease diagnosis and treatment recommendations. The DeepMind Games team further developed AI systems demonstrating superhuman performance in playing Atari games.
DeepMind's adoption of AI localism has propelled it to a prominent position among global AI companies. The company's commitment to ethical AI development and its potential positive impact on the world have garnered acclaim.
Specific instances of DeepMind's AI localism include collaborations with the UK National Health Service (NHS) for diagnosing and treating diseases, with the Royal Society for the Prevention of Cruelty to Animals (RSPCA) for identifying and protecting animals, and with the BBC for creating innovative AI-driven creative content.
Understanding the Role of AI in App Development in the UK
The AI Models Currently in Use
Understanding the fabric of AI localism/globalism requires a working understanding of how AI models use and interpret data.
- Generative AI models utilise existing information and examples to create new, relevant information. An example would be chatbots or virtual assistants that use vast text-based datasets to provide real-time customer support, answer queries, and assist users with tasks.
- Discriminative AI models can classify different objects by identifying patterns in a given data. Mobile apps often use discriminative AI for image recognition tasks, such as identifying objects, people, or scenes in photos. This is commonly found in camera apps, social media platforms, and augmented reality applications.
Interpreting Data
AI understands data by gathering and organising information. It then extracts relevant features and trains models, such as machine learning algorithms or neural networks. These models recognise patterns, enabling predictions or classifications on new data. The interpretative process involves learning from examples, making predictions, and, in some cases, employing reinforcement learning for trial-and-error-based improvement. AI's current capabilities include natural language processing for text, computer vision for images, and speech recognition for auditory data.
Where Does AI Fill in the Gaps?
The scope and capabilities of AI extend to automating or augmenting routine tasks or performing tasks that humans are not capable of doing. Across industries, companies are leveraging this power to reimagine how their business operates.
For example, in healthcare, AI is being used to create customised healthcare plans, while remote health monitoring promotes preventive care and reduced hospitalisations. AI chip and edge computing advancements are fostering more efficient AI processing. Integration of AI with technologies like blockchain and the Internet of Things (IoT) is anticipated to fuel ongoing innovation and growth in the AI industry.
The following table provides a comprehensive breakdown of how different industries are making use of AI models in developing applications.
Industry | Generative AI Applications | Discriminative AI Applications |
---|---|---|
Strategic Planning | Scenario generation for strategic decision-making | Data analysis and pattern recognition for market trends and competitive intelligence |
R&D | Idea generation and concept creation | Predictive modelling for research outcomes and data analysis |
Product Design | Creativity support in design iterations | Image recognition for identifying design elements and user preferences |
Supply Chain | Predictive demand forecasting and optimisation | Object recognition for inventory management and quality control |
Operations | Dynamic process optimisation and automation | Anomaly detection for operational issues and efficiency improvement |
Finance | Financial modelling and risk analysis | Fraud detection, credit scoring, and anomaly detection in transactions |
HR | Automated resume screening and candidate matching | Sentiment analysis for employee feedback and performance evaluation |
IT | Code generation and automated testing | Anomaly detection for cybersecurity and system monitoring |
Legal | Contract drafting and legal document generation | Legal research assistance and contract review using natural language processing |
Marketing & Sales | Content generation for marketing campaigns | Customer segmentation, sentiment analysis, and lead scoring |
Customer Service | Chatbots for customer support and query resolution | Voice recognition for call center interactions and sentiment analysis |
Unlocking the Impact of AI in App Development in the UK
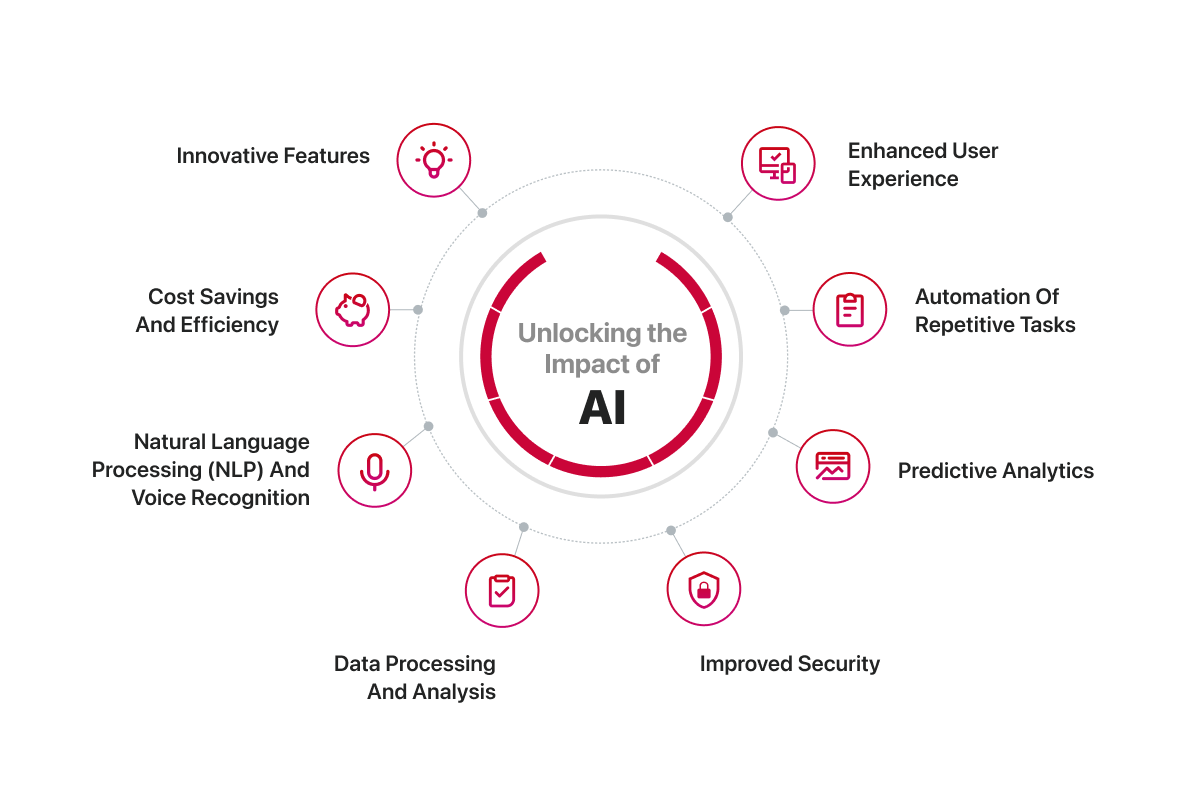
The integration of Artificial Intelligence (AI) in app development has a significant impact on various aspects of the development process as well as the functionality and user experience of the resulting applications.
1. Enhanced User Experience
AI-driven user experiences extend beyond personalisation. Machine learning models can analyse user interactions in real-time, allowing apps to dynamically adjust interfaces, content layouts, and features to optimise user engagement. This adaptability enhances user satisfaction by providing a more fluid and context-aware experience.
2. Automation of Repetitive Tasks
Automation in AI extends to the entire development lifecycle. Beyond code generation and testing, machine learning models can assist in project management, workflow optimisation, and even in the generation of documentation. This comprehensive automation allows developers to focus on higher-level tasks, fostering creativity and innovation.
3. Predictive Analytics
Predictive analytics powered by AI does not only focus on user behaviour but extends to predicting market trends, identifying potential challenges, and suggesting strategies for app improvement. This proactive approach based on data analysis contributes to strategic decision-making and long-term planning.
4. Improved Security
AI enhances security through continuous monitoring and adaptive threat detection. Machine learning algorithms can evolve to recognise new attack patterns and vulnerabilities, providing a more robust defence against emerging cyber threats. Real-time response mechanisms, backed by AI, contribute to a more resilient security posture.
5. Data Processing and Analysis
AI's role in data processing extends to complex data types, such as unstructured data from social media or user-generated content. By employing natural language processing and machine learning algorithms, apps can extract valuable insights from diverse data sources, contributing to a deeper understanding of user sentiment and market dynamics.
6. Natural Language Processing (NLP) and Voice Recognition
Natural language processing goes beyond simple understanding to involve sentiment analysis, context comprehension, and language generation. This sophistication enables the creation of chatbots capable of engaging in more natural and contextually relevant conversations. Additionally, voice recognition technologies are evolving to understand accents, languages, and even emotions, broadening accessibility.
7. Cost Savings and Efficiency
AI's impact on cost savings is not only limited to development processes but extends to resource allocation and infrastructure optimisation. Machine learning algorithms can analyze resource usage patterns, identifying areas for optimisation and cost reduction, leading to more efficient operations.
6. Innovative Features
AI contributes to the development of advanced features like generative models for content creation, recommendation systems that adapt in real-time, and the integration of AI-driven virtual assistants that learn and evolve based on user interactions. These features redefine user expectations and contribute to the overall innovation landscape in app development.
How to Integrate AI and ML in Mobile Applications?
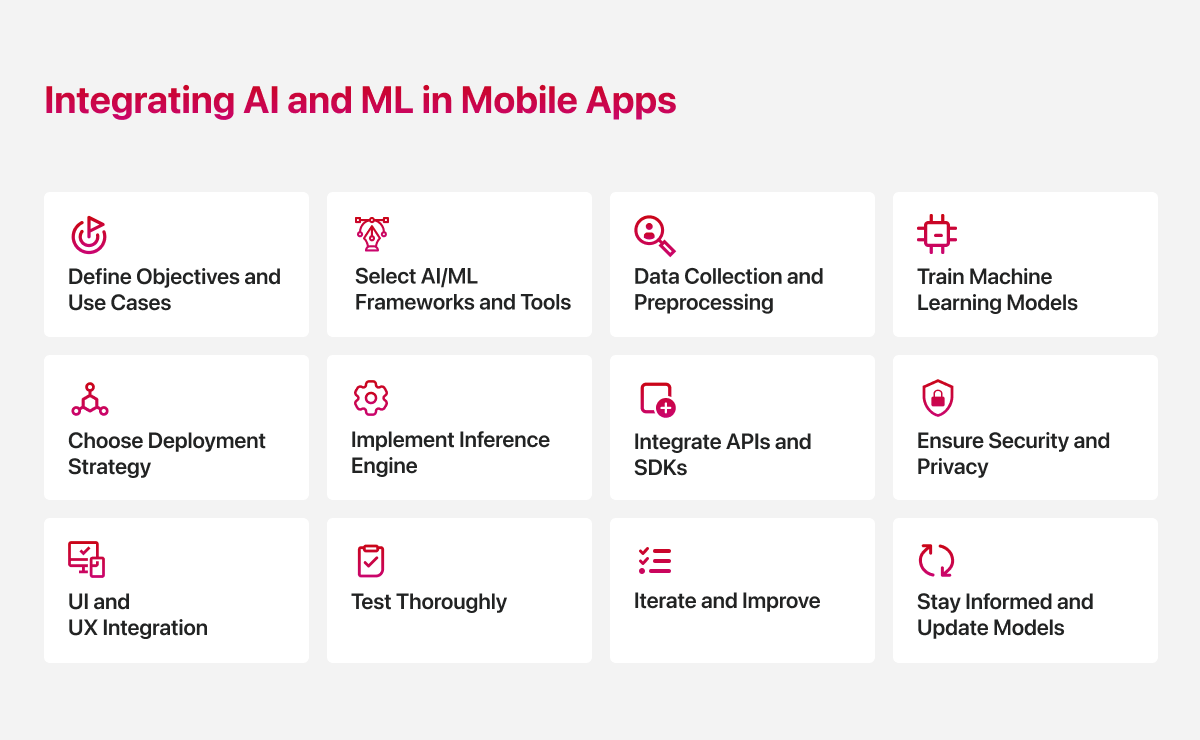
Companies should select between building AI models in-house, fine-tune existing models, or opting for strategic partnerships.
- Building Proprietary Models: Involves creating custom AI models tailored to the specific needs and objectives of the application. This process includes tasks such as defining objectives, selecting frameworks, collecting and preprocessing data, training models, and implementing custom solutions.
- Buying and Fine-Tuning Existing Models: Entails acquiring pre-existing AI models or solutions and fine-tuning them to suit the application's requirements. This approach can save time and resources compared to building models from scratch. Fine-tuning involves adjusting the pre-trained models based on specific data or use cases.
- Strategic Partnerships: Involves collaborating with external AI/ML platforms or service providers through strategic partnerships. This often includes integrating APIs and SDKs from established AI platforms, leveraging their existing models and capabilities. This approach enables applications to benefit from external expertise and resources.
For companies looking to build proprietary models or pursue strategic partnerships, the following is a general guide on how to approach the integration of AI and ML into mobile app development:
1. Define Objectives and Use Cases
This step involves clearly defining the objectives and use cases for integrating AI/ML into the mobile app. Identifying specific problems or areas where AI can enhance user experience, optimise processes, or provide valuable insights is essential.
2. Choose the Right AI/ML Frameworks and Tools
Select suitable AI/ML frameworks and tools based on your project requirements and the expertise of your development team. Popular frameworks include TensorFlow, PyTorch, and scikit-learn for machine learning, and tools like Dialogflow or Wit.ai for natural language processing.
3. Data Collection and Preprocessing
Gather relevant and representative data for training your machine learning models. Ensure that the data is clean, labeled, and appropriately preprocessed. The quality of your data significantly influences the performance of your AI models.
4. Train Machine Learning Models
Use the collected data to train your machine-learning models. Depending on the use case, this might involve supervised learning, unsupervised learning, or reinforcement learning. Regularly evaluate and refine your models to improve accuracy.
5. Choose a Deployment Strategy
Decide whether to deploy your models locally on the mobile device or use a cloud-based approach. On-device deployment is suitable for applications requiring real-time processing, while cloud-based solutions leverage the power of cloud infrastructure.
6. Implement Inference Engine
Develop the inference engine that runs on the mobile device or communicates with the cloud server to make predictions based on the trained models. Optimise the inference process for efficiency, especially in resource-constrained environments.
7. Integrate APIs and SDKs
If you are using pre-trained models or cloud-based services, integrate relevant APIs and SDKs provided by AI/ML platforms. This simplifies the integration process and allows you to leverage existing AI capabilities.
8. Ensure Security and Privacy
Implement security measures to protect sensitive user data, especially if your app deals with personal information. Ensure compliance with privacy regulations and standards. If using cloud services, make sure data transmission is encrypted.
9. User Interface (UI) and User Experience (UX) Integration
Design an intuitive and user-friendly interface that seamlessly integrates AI features. Communicate AI-driven insights or actions clearly to users. Consider how AI enhancements fit within the overall user experience.
10. Test Thoroughly
Conduct comprehensive testing of your AI/ML integration. Test for accuracy, reliability, and performance in different scenarios. Pay attention to edge cases and ensure that the AI features enhance, rather than hinder, the user experience.
11. Iterate and Improve
Monitor the performance of your AI models post-launch. Collect user feedback and use analytics to understand how users interact with the AI features. Iterate and improve your models based on real-world usage.
12. Stay Informed and Update Models
Keep abreast of advancements in AI/ML technology. Periodically update your models to incorporate new data, improve accuracy, and adapt to changing user behaviours or industry trends.
How Much Does it Cost to Develop an AI-powered App in the UK?
AI App Complexity | Development Time (Hours) | Estimated Cost (£) | Examples |
---|---|---|---|
Basic | 300-500 | £15,000 - £40,000 | Simple chatbots, Basic recommendation engines |
Intermediate | 500-800 | £25,000 - £80,000 | Personalised content recommendation apps, Facial recognition in apps |
Advanced | 800+ | £40,000 - £100,000 or more | Natural language processing-based virtual assistants, Complex healthcare diagnosis apps |
Some Real-world Examples of Apps Using AI Technology
For companies, AI-driven disruptions are no longer an ambiguous concept but a hard reality. In such circumstances, staying ahead of the curve would mean incorporating AI technology into business processes. From developing novelty products to gaining loyal customers, early AI implementation is not without benefits for businesses.
Progressive enterprises are leveraging AI to drive inventive customer products and experiences across various sectors. A notable example is JPMorgan in financial services, where they are crafting an AI model to assist customers in choosing investments customised to their unique circumstances and preferences. Meanwhile, in the realm of sports, the NBA stands out with its array of AI initiatives, introducing innovations like personalised highlight reels that transform the basketball fan experience.
Some other companies making use of AI are listed below.
Netflix
The streaming platform adjusts content recommendations and user interfaces in real-time based on viewing habits and preferences.
Jira by Atlassian
A project management tool that automates task assignment, workflow processes, and offers integrations for comprehensive project automation.
Google Assistant
A virtual assistant that utilizes NLP and voice recognition for natural language understanding and voice-activated commands.
AWS Cost Explorer
An app within Amazon Web Services that uses machine learning to analyze resource usage, identify cost-saving opportunities, and optimize infrastructure efficiency.
Spotify
The music streaming app employs recommendation algorithms that adapt in real-time based on user listening habits and preferences.
Future Vision and Current Paradox
Navigating AI Uncertainties
In spite of the plethora of opportunities AI offers, the technology is not without its own set of challenges. Local and global issues such as cost barriers, lack of complete data, inaccuracy in predictions, potential vulnerabilities in cybersecurity, and concerns related to safeguarding data privacy are some pressing problems that remain to be addressed. While cybersecurity threats pose risks to the integrity and security of AI systems, inaccurate AI outputs from incomplete data can lead to unreliable decisions.
The collection and use of vast amounts of personal data raise concerns about privacy breaches, emphasising the need for robust measures to address these challenges in the development and deployment of AI technologies.
While the UK’s 2023 AI Safety Summit led to the signing of the Bletchley declaration, focussed on addressing the risks AI poses and a multilateral agreement to test advanced AI models, developments on AI regulation both on local and global levels remain to unfold.
Staying Relevant
With AI becoming an integral part of business operations and consumer-facing applications, businesses should be poised to take charge of the disruptive changes the technology will bring with it. The gradual access to big data, combined with advancements in cloud computing infrastructure and a demand for automation are enabling a range of novel opportunities for AI applications in every industry.
In this landscape, AI localism and globalism are meant to combine into a collaborative model, setting the path for reshaping industries.