Table of Contents
Deep Learning and Neural Networks Enabling AI in Healthcare
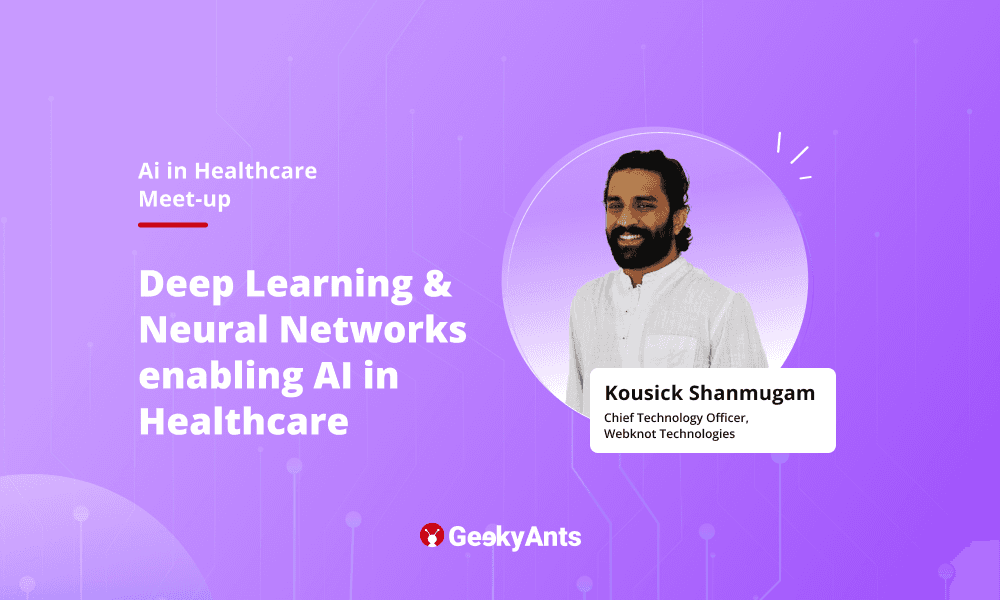
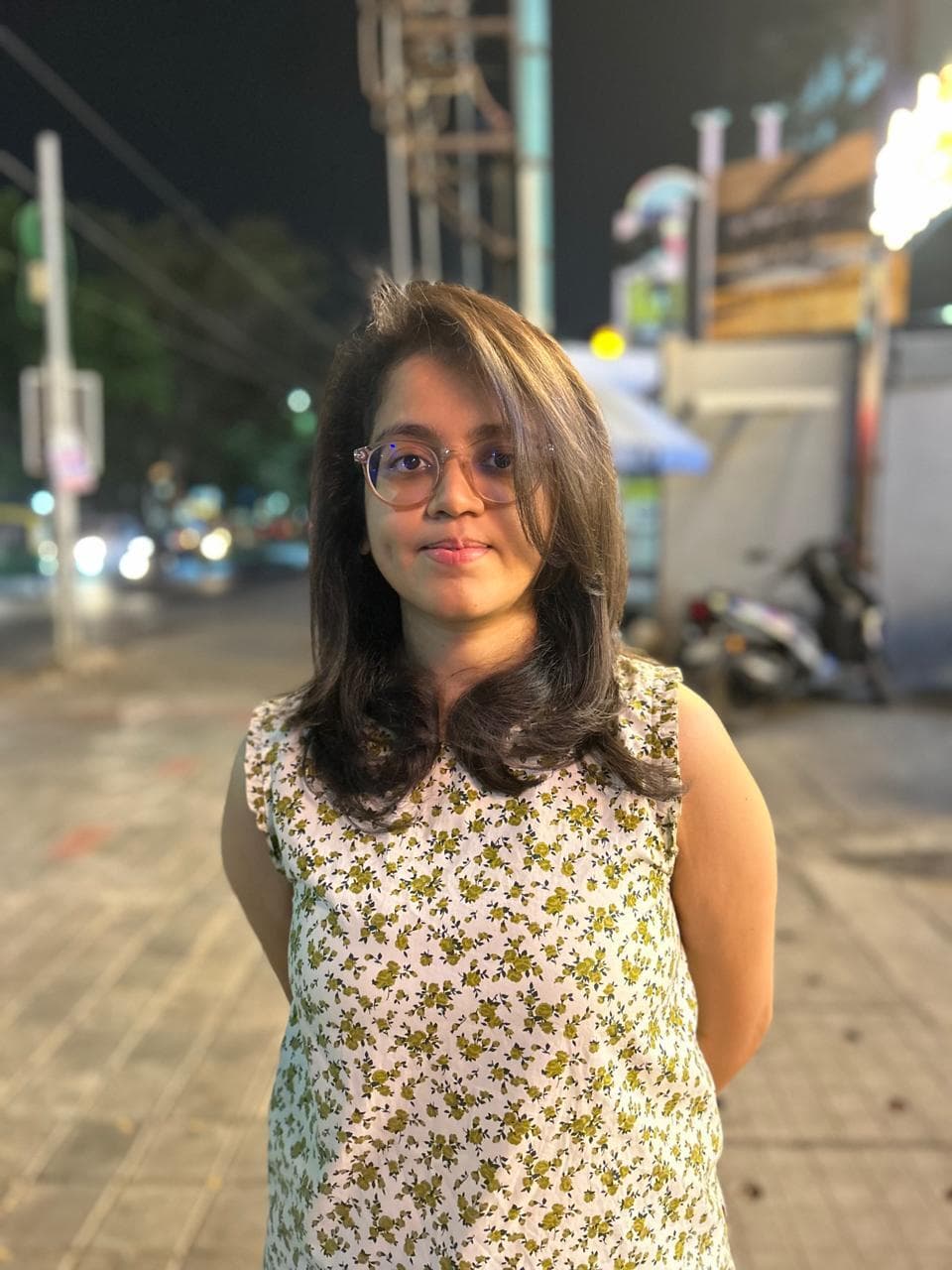
Book a call
In the ever-evolving landscape of technology, the fusion of deep learning, neural networks, and artificial intelligence has paved the way for groundbreaking advancements in various industries. This blog explores the profound impact of deep learning and neural networks, particularly in the realm of healthcare. Let us dive into the key elements that have fueled the growth of this revolutionary technology and understand how it is transforming the healthcare sector.
The Growth Trajectory of Deep Learning
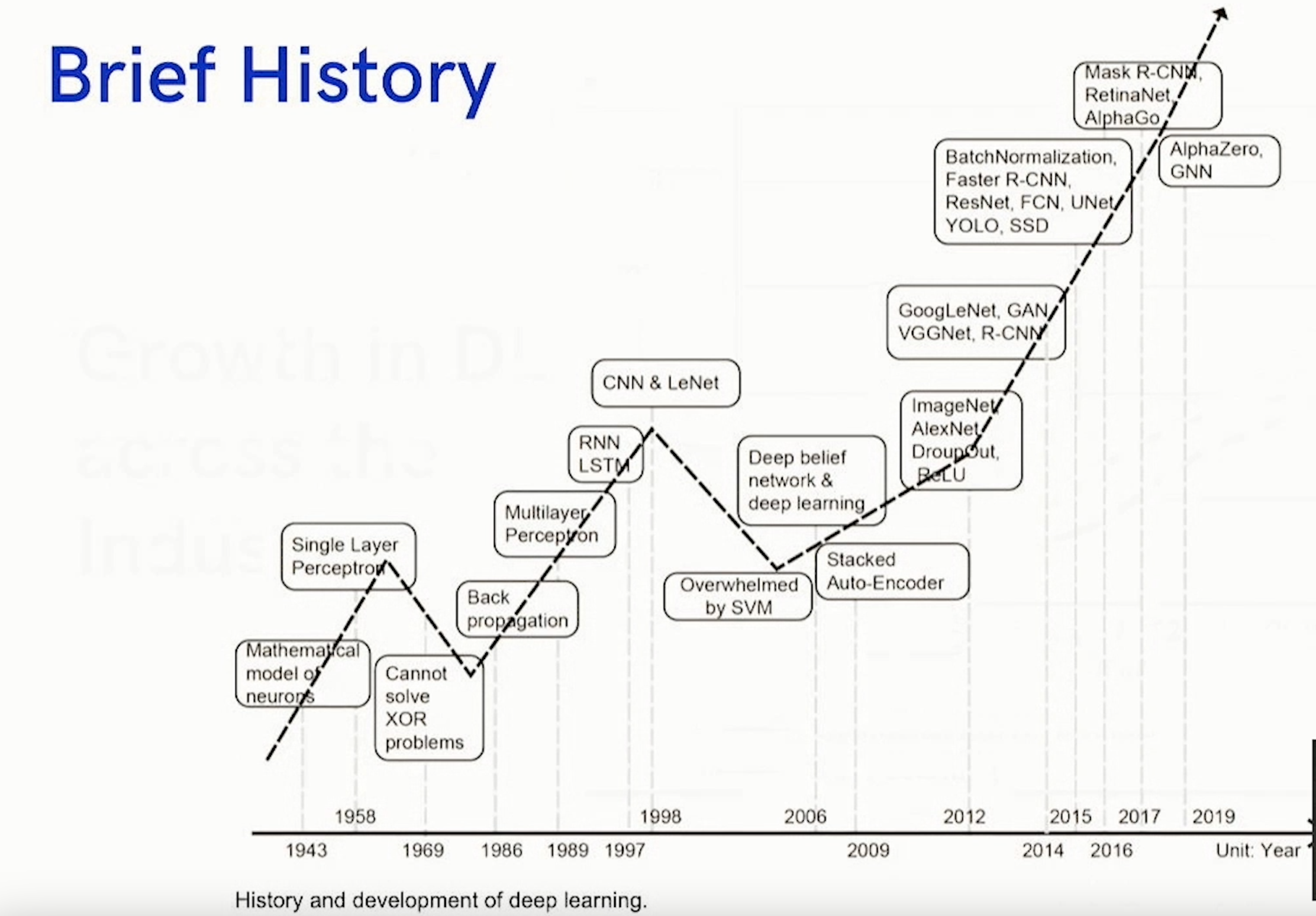
The journey of deep learning has witnessed a remarkable surge, as depicted in the industry's growth graph. The pivotal factor contributing to this surge is the unprecedented increase in compute power. Cloud computing, pioneered by major players like AWS and GCP, has played a pivotal role in unleashing the potential of deep learning algorithms. The availability of massive computational resources has allowed researchers and data scientists to translate theoretical concepts from research papers into practical solutions.
The proliferation of research papers and publications, especially in deep learning and machine learning, serves as a testament to the industry's growth. The surge in scholarly contributions on platforms like Google Scholar reflects the diverse range of challenges being addressed by professionals worldwide. The combination of abundant data, enhanced storage capacity, and advanced instruments has propelled deep learning and machine learning into real-world implementations.
Evolution of Neural Networks
Delving into the roots of neural networks, we trace their origins back to 1943 when the concept of deep learning emerged. Initially introduced in research papers, it gained traction with the development of the perceptron by Frank Rosenblatt in 1957. The subsequent evolution led to the advent of Convolutional Neural Networks (CNN) in 1998, marking a breakthrough in computer vision. Further milestones include the introduction of AlexNet in 2012 and GoogleNet, both of which elevated the capabilities of neural networks in image analysis.
The historical context underscores that deep learning is not a recent phenomenon. Instead, it has been an ongoing journey, progressing from theoretical concepts to practical implementations. Today, large language models like GPT demonstrate the continuous innovation in the field, with researchers pushing the boundaries of what neural networks can achieve.
Understanding Deep Learning
At its core, deep learning seeks to mimic the human brain's functioning through artificial neural networks. These networks comprise an input layer, hidden layers, and an output layer. The process involves perceiving input, passing it through hidden layers of interconnected neurons, and producing an output – mirroring the human brain's ability to interpret sensory information and generate responses.
Distinguishing deep learning from traditional machine learning, the former exhibits a self-learning capacity. While machine learning relies on explicit feature extraction, deep learning leverages intricate mathematical equations, weights, and activation functions to autonomously discern patterns from vast datasets. This inherent intelligence enables deep learning models to make nuanced classifications, even when specific labels may be ambiguous.
Components of a Neural Network
A neural network consists of an input layer, multiple hidden layers, and an output layer. The hidden layers serve as intermediaries, processing and assigning weights to various features of the input data. Activation functions, such as linear, sigmoidal, and rectified linear unit, determine the flow of information between neurons. The complexity of deep learning increases with the depth of these hidden layers, a characteristic that defines the depth of neural networks.
Training neural networks involves iterative processes like feed-forward and backward propagation. Feed-forward entails the initial prediction of output, while backward propagation adjusts weights and activation functions based on the disparity between predicted and actual outcomes. The continuous refinement through training data enhances the network's ability to generalize patterns and make accurate predictions.
Deep Learning in Healthcare: A Critical Need
The integration of deep learning and neural networks in healthcare addresses significant challenges faced by the industry. A global shortage of expert radiologists and pathologists necessitates technological interventions to bridge the gap in diagnostic capabilities. The advent of cloud computing, coupled with the abundance of health data, provides a fertile ground for developing tools that expedite disease identification and streamline healthcare processes.
The wealth of patient health records and advancements in diagnostic tools present opportunities for predictive analytics and enhanced diagnostic accuracy. Two prominent neural network architectures, Convolutional Neural Networks (CNN) and Recurrent Neural Networks (RNN), exemplify the transformative applications of deep learning in healthcare.
Convolutional Neural Networks (CNN) in Healthcare
CNN, renowned for its proficiency in image analysis, has demonstrated unparalleled accuracy in diagnosing medical conditions. Leveraging datasets containing diverse medical images, CNNs excel in classifying and interpreting complex visuals. A notable case study involves Google's collaboration with Indian hospitals to address diabetic retinopathy, showcasing CNN's ability to analyze fundus images and predict disease progression with remarkable accuracy.
Recurrent Neural Networks (RNN) for Clinical Predictions
In the realm of clinical predictions, Recurrent Neural Networks (RNN) exhibit prowess in analyzing sequential data. A notable study by Jimmy's son and team illustrates the use of RNN to predict clinical events based on patients' previous health records. This approach surpasses traditional methods, achieving over 70% accuracy in predicting future clinical outcomes.
The Future Landscape of Healthcare
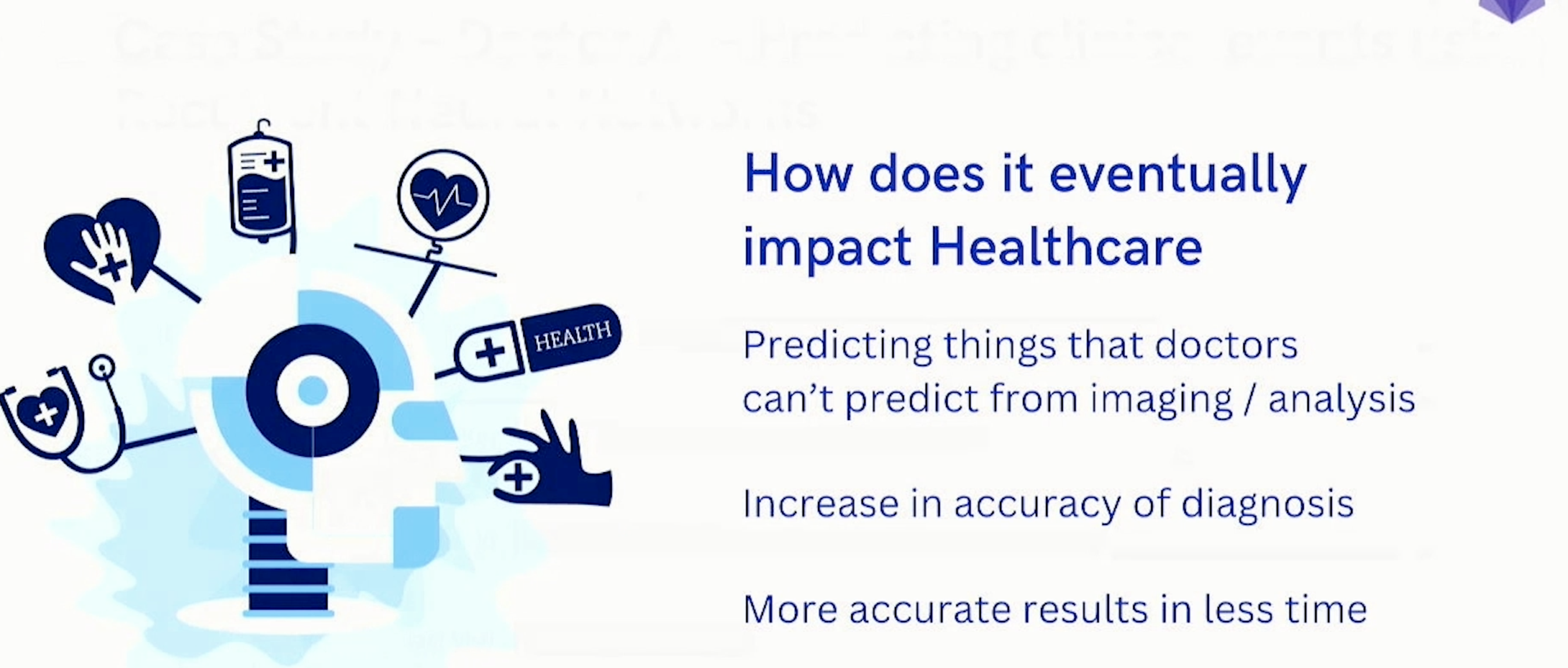
The integration of deep learning and neural networks in healthcare heralds a new era of predictive analytics, personalized treatments, and accelerated diagnostic processes. These technologies empower healthcare professionals to make informed decisions, particularly in regions facing a scarcity of medical experts.
As we navigate the intersection of technology and healthcare, the transformative impact of deep learning and neural networks continues to unfold. From image analysis to predictive modeling, these innovations contribute to a more efficient, accurate, and accessible healthcare ecosystem. The ongoing research and real-world implementations underscore the boundless potential of artificial intelligence in revolutionizing healthcare practices.
Looking Ahead
The journey from theoretical foundations to practical applications has firmly established deep learning and neural networks as indispensable tools in the healthcare sector. Embracing these technological advancements is not merely an option but a necessity for fostering a healthier and more resilient global community.
You can check out the entire talk here 🔽
Dive deep into our research and insights. In our articles and blogs, we explore topics on design, how it relates to development, and impact of various trends to businesses.