Table of Contents
AI-Driven Anomaly Detection in Healthcare Enhancing Diagnostics & Efficiency
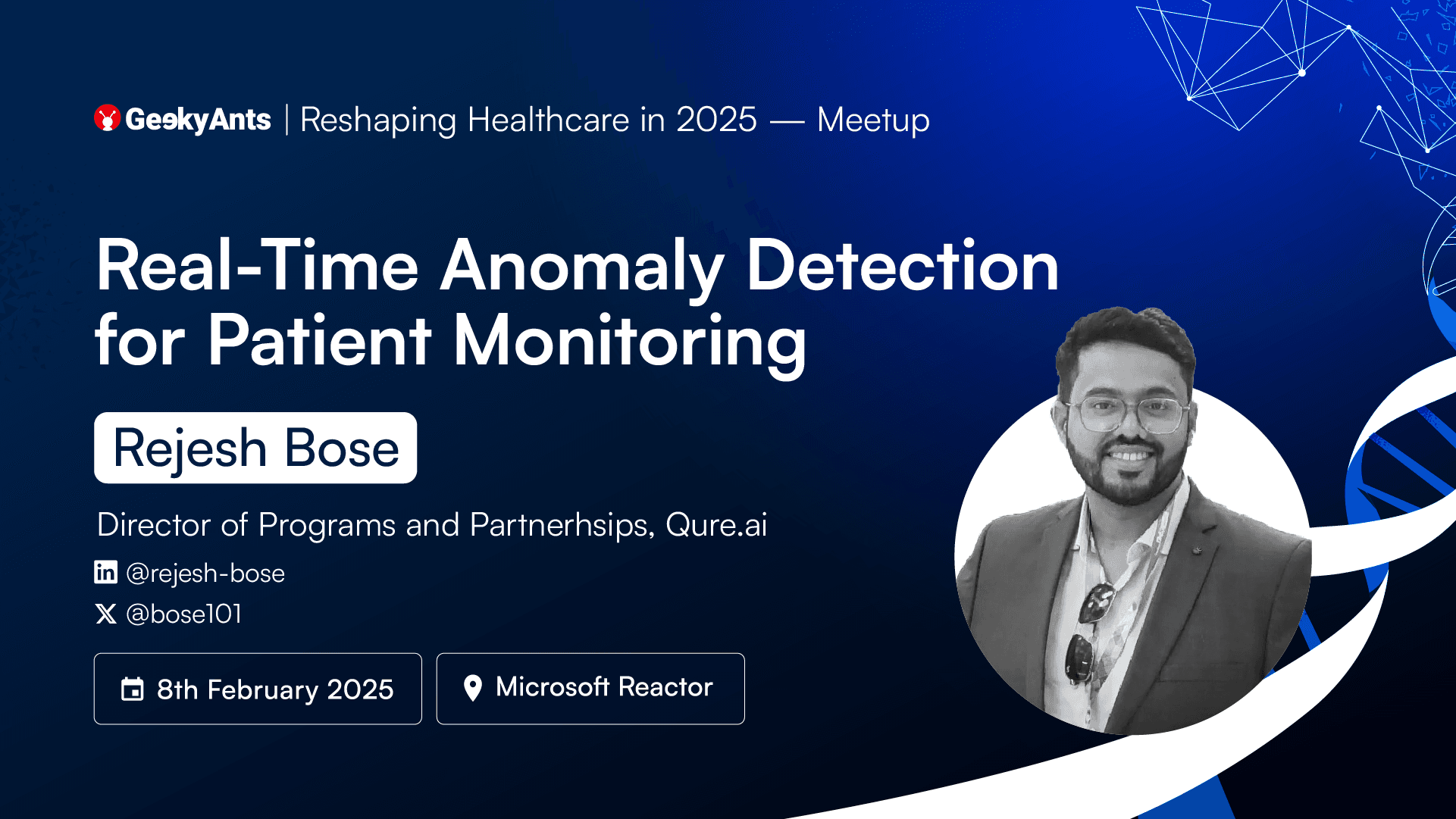
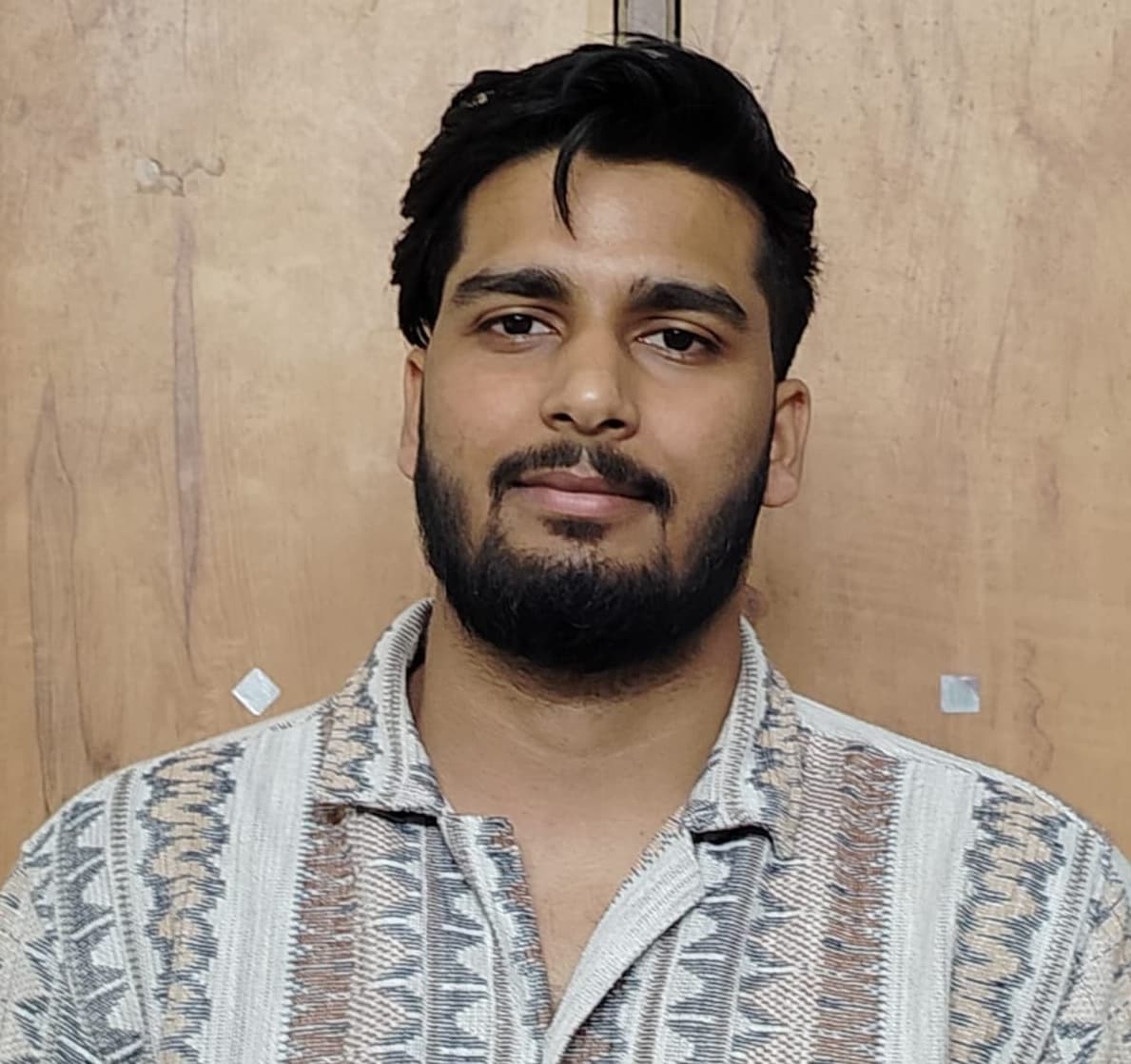
Book a call
Editor’s Note: This blog is an adapted transcript of Rajesh Bose’s talk at the GeekyAnts Healthcare AI Meetup. Rajesh, Director of Programs at Qure.ai, shared insights on the role of AI in anomaly detection within healthcare. While refined for clarity and brevity, this transcript captures the depth and essence of the session.
AI-Driven Anomaly Detection in Healthcare
Hello everyone! My name is Rajesh Bose, and I work at Qure.ai as a Director of Programs. Now that the tone has been set, I think you all understand—I am not a core tech guy, but I rely on the tech team for support. Similarly, at Qure.ai, I am part of the operations team, where my role is to streamline operations across multiple countries and healthcare systems.
Qure.ai is an AI-powered radiology company focusing on oncology, lung cancer screening, tuberculosis detection, and other diseases through medical imaging. Our AI models analyze chest X-rays and brain CT scans to identify anomalies. Today, I’ll be talking about anomaly detection and its use cases in healthcare, but before that, let me briefly introduce what we do as a company.
Currently, we operate in over 90 countries, which means we have obtained 90 different regulatory clearances. Our AI solutions are deployed in some of the most remote regions across Latin America, Africa, and beyond, impacting over 25 million lives. In the U.S. alone, we hold 18 FDA clearances and have AI tools installed at over 3,000 healthcare facilities, including hospitals and screening centres.
Our business model is simple—our AI integrates seamlessly with X-ray and CT consoles in hospitals. For instance, when working with NHS UK, our AI solutions are deployed in hospitals under a contractual agreement, screening for specific diseases based on the hospital’s requirements. While our product can detect 30+ diseases, we typically tailor it to a few key use cases to ensure streamlined operations.
We have a robust team of 300-350 professionals, including 100-150 AI engineers. Our AI model is one of the few recommended by the World Health Organization for use in settings where radiologists are unavailable. This enables healthcare providers to detect tuberculosis in remote areas without access to radiologists.
Understanding Anomaly Detection in Healthcare
Anomaly detection is straightforward—it refers to identifying unusual patterns within data. In simple terms, if a person has an irregularly high heart rate, AI can detect that deviation from the normal range. This concept applies across industries, from banking fraud detection to healthcare diagnostics.
In banking, for example, if you typically spend small amounts on transactions and suddenly make multiple large purchases in a short span, your bank may flag these as anomalies and trigger security alerts. Similarly, in healthcare, AI can detect deviations in patient vitals, medical imaging, and hospital equipment data, providing valuable insights to healthcare providers.
However, the regulatory landscape in healthcare makes AI implementation more complex. Unlike financial fraud detection, where banks can instantly notify users of suspicious transactions, healthcare anomaly detection must be carefully managed. AI cannot simply send an alert stating, “You’re about to have a heart attack—seek medical attention immediately.” Instead, notifications must be controlled, relayed through authorized medical professionals, and structured in a way that aligns with regulatory guidelines.
Types of Anomalies in Healthcare
There are different types of anomalies AI can detect:
- Point anomalies: These occur when a single data point deviates from the norm, such as a sudden spike in heart rate or glucose levels.
- Contextual anomalies: These are abnormal readings that are context-dependent. For example, a sudden drop in temperature might be normal in winter but unusual in summer.
- Collective anomalies: These occur when a group of data points together form an anomaly, such as repeated equipment malfunctions in a hospital setting.
AI Applications in Medical Imaging and Diagnostics
One of the most impactful applications of AI anomaly detection is in medical imaging. AI models analyze X-rays, CT scans, and MRIs to detect early signs of lung nodules, tuberculosis lesions, and brain swellings. Since medical imaging patterns remain consistent across different populations, AI can effectively be trained to recognize these anomalies and assist radiologists in diagnosis.
At Qure.ai, our AI systems are trained on vast datasets, enabling them to flag abnormalities in medical scans for further review by radiologists. However, despite its advancements, AI in healthcare must comply with strict regulations. Any flagged anomaly must be reviewed by a licensed medical professional before any diagnosis or treatment plan is suggested.
AI in Hospital Operations and Efficiency
Beyond diagnostics, AI-driven anomaly detection improves hospital operations. Hospitals deal with complex workflows, administrative overload, and resource allocation challenges. AI helps identify inefficiencies in emergency room admissions, patient discharge processes, and hospital supply chains.
For example, AI can analyze patient admissions to detect spikes in disease outbreaks within specific geographic areas. If a sudden increase in flu cases is observed from a particular neighbourhood, hospitals can prepare accordingly by allocating more resources. Similarly, AI can help prevent insurance fraud by analyzing claim patterns and flagging suspicious activity.
Future of AI in Healthcare Anomaly Detection
Looking ahead, AI’s role in anomaly detection will continue to expand. Future applications include:
- Genomic Data Analysis: AI will help detect genetic anomalies, enabling early diagnosis of hereditary diseases.
- Speech and Behavioral Anomaly Detection: AI will analyze speech patterns and behaviors to detect cognitive decline and mental health conditions.
- Pandemic Prediction Models: AI-driven models will analyze real-time data to predict disease outbreaks and help healthcare systems respond proactively.
Final Thoughts
AI anomaly detection is revolutionizing healthcare by enabling early disease detection, optimizing hospital operations, and improving predictive analytics. However, regulatory challenges must be carefully managed to ensure ethical AI implementation. As technology evolves, healthcare organizations must embrace AI’s potential while maintaining human oversight.
For those interested in working with us at Qure.ai, we are always open to collaboration. If you are passionate about AI in healthcare, reach out—we’d love to hear from you. Thank you!
Dive deep into our research and insights. In our articles and blogs, we explore topics on design, how it relates to development, and impact of various trends to businesses.