Table of Contents
Why Businesses Need Explainable AI - and How to Deliver It
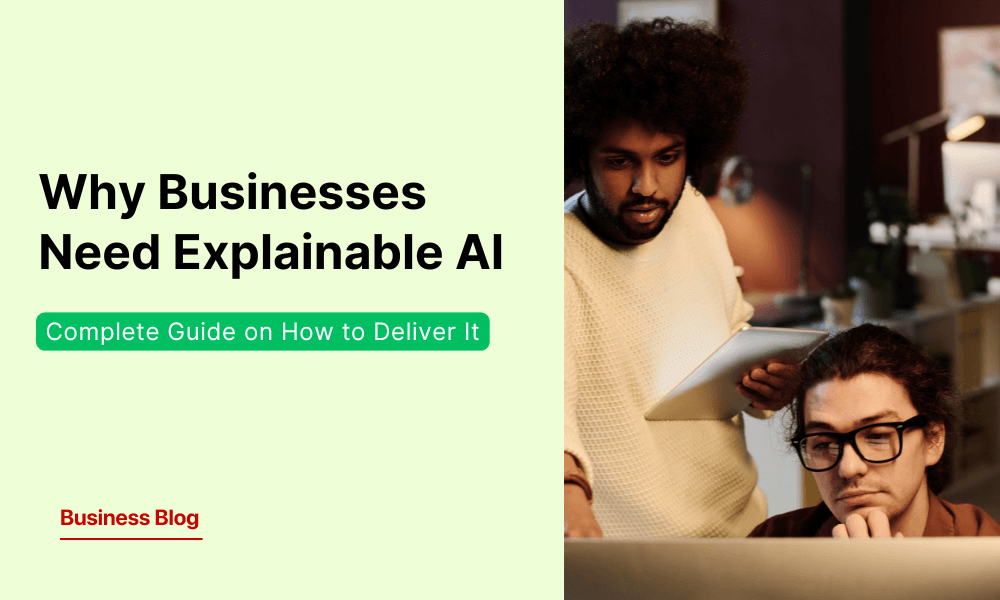
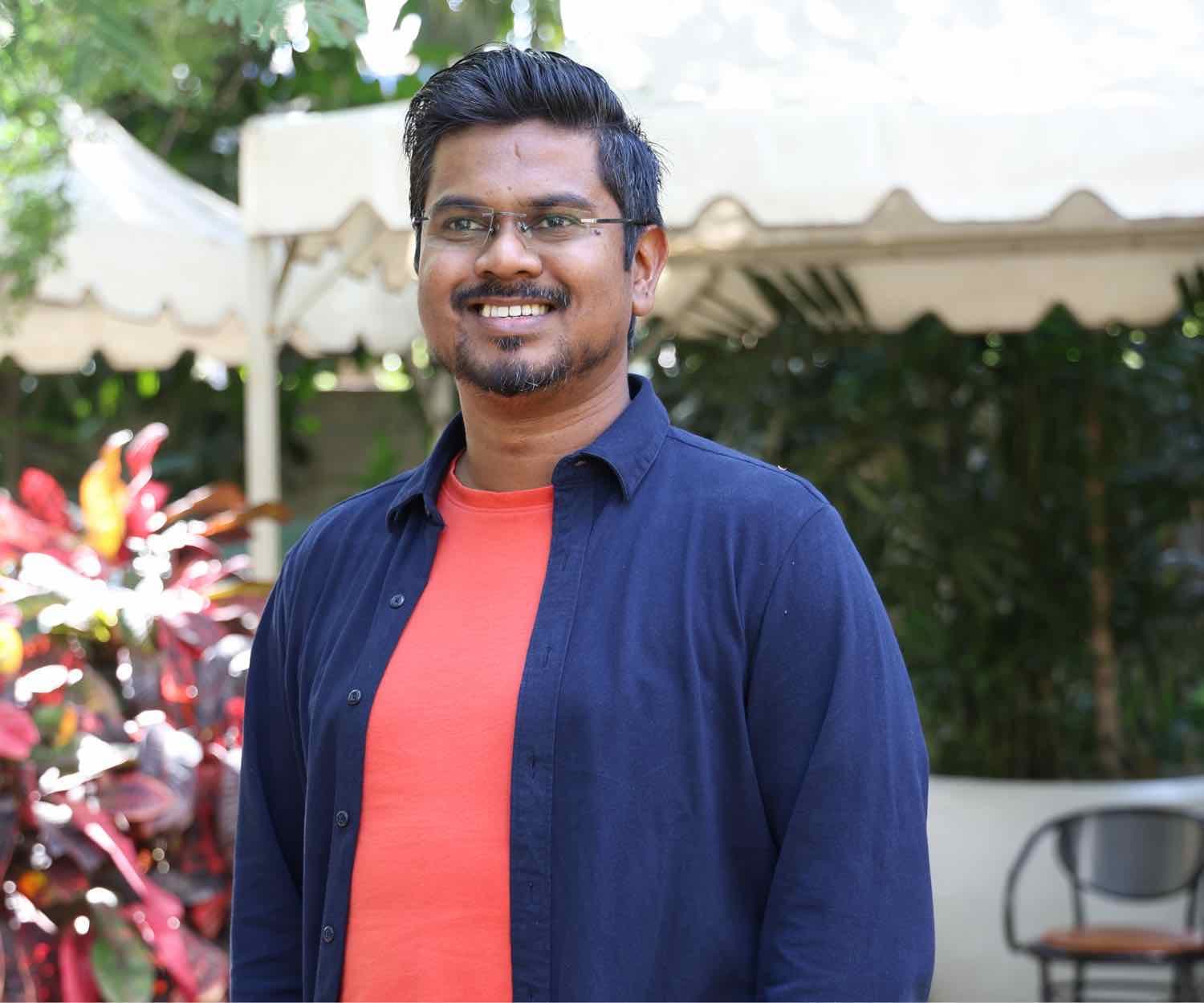
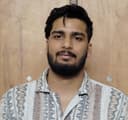
Book a call
Every AI decision—whether approving a loan or flagging a health risk—carries consequences. But when those decisions lack explanation, they raise more questions than answers—fueling mistrust, regulatory scrutiny, and operational risk.
The real challenge? Many AI systems deliver outputs without showing their reasoning. This creates a critical blind spot for businesses—especially in industries where accountability is not optional.
Explainable AI (XAI) closes that gap. By turning opaque outputs into clear, auditable logic, XAI empowers organizations to validate decisions, meet compliance requirements, and build trust across teams and customers.
Explainability has become a strategic necessity with the XAI market projected to surpass $21 billion by 2030. In this blog, we explore why it matters—and how to deliver it effectively.
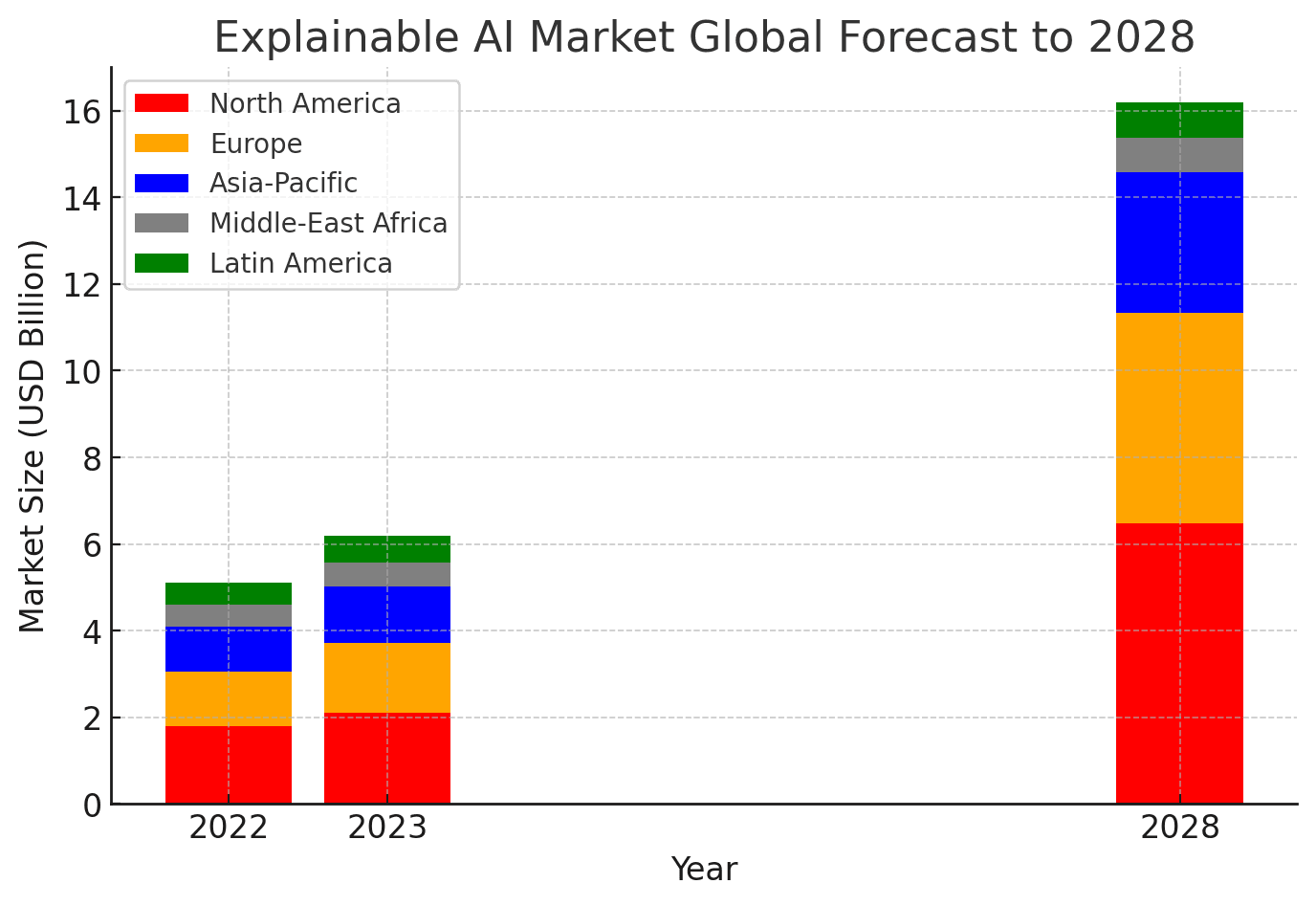
What is Explainable AI (XAI)?
Explainable AI (XAI) is a set of techniques that make AI decisions transparent and understandable to humans. Instead of treating AI as a black box, XAI provides insight into how models arrive at specific outcomes—essential in industries where decisions carry real consequences.
For example, if an AI system denies a loan, XAI can show that the decision was based on low income and poor credit history—helping both users and auditors understand the logic.
Principles of XAI
Core principles include transparency, interpretability, fairness, and accountability—ensuring models can be trusted, validated, and improved.
AI vs. XAI
Aspect | Traditional AI | Explainable AI (XAI) |
Decision Transparency | Opaque (“black box”) | Transparent and interpretable |
User Understanding | Hard to understand how decisions are made | Provides clear reasoning behind outcomes |
Trust & Accountability | Low trust due to lack of clarity | Builds trust through explainable outputs |
Regulatory Compliance | Difficult to audit | Supports compliance with interpretable logic |
Use Case Fit | Optimized for accuracy and performance | Balanced with interpretability for high-stakes decisions |
Stakeholder Engagement | Limited to technical teams | Enables collaboration across business units |
Why Explainable AI Is Important for Your Business
Every AI-powered decision carries weight—but without clarity, that weight turns into risk. Whether it is denying a loan, diagnosing a condition, or recommending a course of action, AI systems that can not explain their reasoning create blind spots for businesses and stakeholders alike.
The core problem lies in opacity. When models deliver results without context, businesses face serious consequences: compliance violations, user distrust, operational delays, and unchecked algorithmic bias. In sectors like finance and healthcare, a lack of transparency is not just problematic—it can be unlawful. Regulations such as GDPR (Article 22) and the upcoming EU AI Act explicitly require automated decisions to be explainable, ensuring fairness, accountability, and auditability at scale.
Explainable AI (XAI) addresses this head-on. Revealing the logic behind AI decisions helps organizations:
- Comply with regulatory frameworks and avoid penalties.
- Strengthen user trust by making outcomes interpretable and fair.
- Enable internal audibility to catch bias and errors before they scale.
- Support ethical governance in high-impact environments.
More importantly, XAI fosters confidence among non-technical stakeholders—executives, clients, and customers—by making AI systems more relatable and defensible. In a time when digital trust is becoming a differentiator, explainability isn’t a backend technical feature—it is a competitive advantage.
Benefits
1. Improves Decision Transparency
XAI reveals the reasoning behind AI outputs, helping teams validate predictions, spot inconsistencies, and prevent blind acceptance of flawed outcomes.
2. Enables Regulatory Compliance
In sectors like finance and healthcare, regulations now demand explainability. XAI offers traceable, audit-ready logic that satisfies GDPR, HIPAA, and other compliance frameworks.
3. Builds Trust Across Stakeholders
Whether it’s a compliance officer, business leader, or customer—everyone can see why a decision was made. This visibility increases confidence, reduces friction, and accelerates adoption.
4. Bridges the Technical–Business Divide
Interpretable results promote collaboration between engineers, analysts, and non-tech teams—aligning decisions with business goals.
5. Accelerates Model Debugging and Optimization
XAI pinpoints what went wrong when AI outputs fail. Developers can trace issues back to inputs, retrain models faster, and ensure ethical accuracy.
6. Drives Adoption and ROI
Teams use what they understand. With XAI, AI tools are more likely to be trusted, deployed, and scaled, maximizing business value.
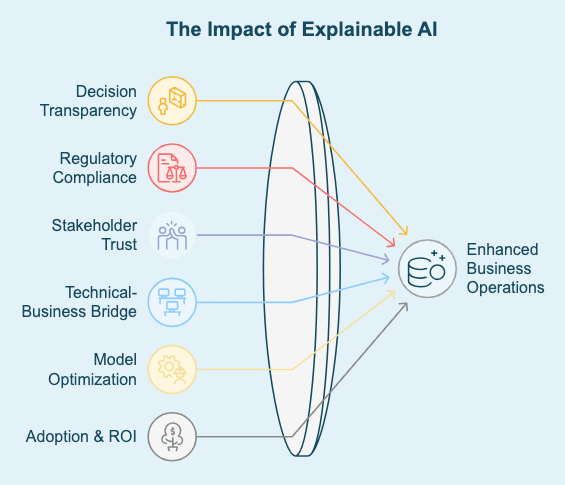
In essence, explainability turns AI from a black box into a business ally—trusted, compliant, and optimized for impact.
How Does Explainable AI Work?
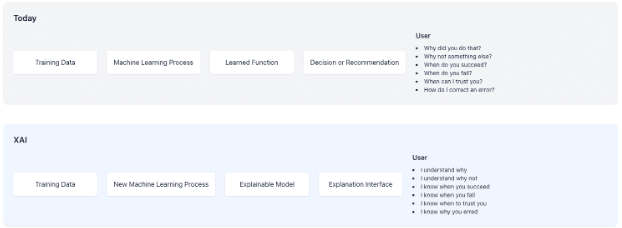
Explainable AI (XAI) works by translating complex model behavior into human-understandable insights. It does this in two ways: by using interpretable models from the start or by layering explanation techniques onto black-box models like neural networks or ensemble algorithms.
Here’s how it works under the hood:
1. Interpretable (White-Box) Models
Some algorithms—like decision trees, rule-based systems, and linear regression—are inherently explainable. Their outputs can be directly traced back to specific input conditions. These models are ideal when clarity outweighs the need for high predictive complexity.
Use case: Regulatory environments where traceability is essential, even at the cost of slight performance trade-offs.
2. Feature Importance Analysis
Tools like SHAP (SHapley Additive Explanations) and LIME (Local Interpretable Model-Agnostic Explanations) rank input features by their influence on predictions.
- SHAP assigns a consistent contribution value to each feature—based on game theory—to explain how much it moved the result up or down.
- LIME creates a simple model near the prediction instance to simulate the black-box behavior locally.
Example: A credit scoring model might show that “low income” contributed 40% and “high debt ratio” 35% to a loan rejection—turning the output into a defensible business rationale.
3. Local Explanation Methods (LIME, SHAP)
These techniques focus on individual predictions:
- LIME helps interpret a single decision by approximating the complex model with an understandable one.
- SHAP gives an additive breakdown of each feature’s effect on a single result.
These methods are especially useful in high-stakes domains where each AI output must be reviewed or justified case by case.
4. Visual Explanations
Visual tools like heatmaps, feature attribution graphs, and partial dependence plots help teams see what the model focused on—across image, tabular, and text data.
Example: In image recognition, a heatmap can highlight specific regions that led to a diagnosis. In NLP, word importance maps show why a sentence was flagged for sentiment or intent.
5. Natural Language Explanations
Some systems go a step further by converting model outputs into plain English summaries.
Example: Instead of just showing a chart, the system may say: “The loan was denied due to low income and short credit history, which are linked to high default rates in similar profiles.”
This brings non-technical users—executives, customers, auditors—into the loop.
6. What-If and Counterfactual Analysis
Advanced XAI tools can simulate alternative scenarios to show how changing one input would alter the output.
Example: “Had the applicant’s income been $10,000 higher, the loan would have been approved.”
This helps in identifying thresholds, improving fairness, and refining decision policies.
7. Built-In Platform Capabilities
Platforms like Google Vertex AI, IBM Watson OpenScale, and Microsoft InterpretML offer built-in XAI toolkits. These generate explanation dashboards, detect model bias, and provide compliance-ready logs—reducing the burden of building explainability from scratch.
In summary, XAI combines statistical techniques, user-friendly outputs, and platform-driven tools to ensure AI decisions are clear, defensible, and business-ready—turning machine intelligence into trusted intelligence.
Enterprise Adoption: How Tech Giants Are Enabling XAI
Major AI platforms have already integrated explainability into their ecosystems—making it easier for businesses to adopt XAI without starting from scratch.
IBM Watson OpenScale offers built-in explainability tools that monitor AI models in real-time. It quantifies which input features drove specific predictions and flags potential bias. By combining explainability with AI governance, IBM helps enterprises maintain trust and accountability at scale.
Google Vertex AI Explainability assigns influence scores to each input, showing how much each feature contributed to a prediction. For example, in an image classified as a “cat,” the model might reveal that 40% of the decision was based on whisker patterns and 30% on ear shape. Google emphasizes that in regulated sectors, AI without interpretability is “out of bounds,” highlighting explainability as a non-negotiable feature.
These enterprise-grade tools accelerate XAI adoption and ensure that AI decisions remain transparent, auditable, and aligned with compliance requirements.
How Do You Adopt Explainable AI (XAI) into Your Business?
Integrating Explainable AI into your organization is not a plug-and-play decision—it requires a strategic, step-by-step approach aligned with your business goals, stakeholders, and industry context.
1. Define Business Objectives and Compliance Needs
Start by identifying why you need explainability. Is it to meet regulatory standards, enhance stakeholder trust, or debug complex models?
Example: A bank may focus on credit decision transparency, while a healthcare provider may need physician-readable outputs for patient-facing models.
2. Choose Models with Interpretability in Mind
If the use case allows, opt for inherently interpretable models like decision trees or linear regression. When using complex models (e.g., deep learning), plan early for an explainability layer using tools like SHAP or LIME.
Tip: Do not bolt on explainability—bake it into your model design phase.
3. Leverage Existing Tools and Platforms
Use proven XAI solutions to accelerate adoption and reduce development overhead.
- IBM Watson OpenScale provides real-time model monitoring and bias detection.
- Google Vertex AI delivers feature attribution and influence scores out of the box.
- Open-source libraries like SHAP, LIME, and Microsoft’s InterpretML integrate easily into most ML workflows.
Note: Look for industry-specific tools in regulated sectors like fintech and healthcare for maximum compliance alignment.
4. Design User-Centric Explanation Interfaces
Explainability only adds value when the intended audience can understand it. Design outputs for clarity—dashboards for analysts, plain-text summaries for customers, and audit logs for compliance teams.
Example: A chatbot could offer a “Why did I get this result?” prompt, while internal reports might include detailed feature weights.
5. Monitor, Validate, and Improve
XAI is an ongoing practice, not a one-time implementation. Regularly assess both the AI’s decisions and the quality of the explanations.
Ask:
- Are explanations aligned with the model's logic?
- Are users finding them useful and understandable?
- Are explanations exposing bias or flawed logic?
Continuously refine your models and explanation strategies based on feedback, and perform regular audits to ensure long-term transparency and compliance.
By following this structured approach—starting with goals, selecting the right models, using the right tools, and ensuring interpretability for real users—you can embed explainability into your AI initiatives from the ground up. Start small, iterate, and scale with confidence.
Challenges in Adopting Explainable AI
Explainable AI offers clarity—but implementing it comes with its own set of complexities. Here are the key challenges businesses must navigate:
1. Accuracy vs. Interpretability Trade-off
Highly accurate models like deep neural networks are often the least transparent. Simpler models are easier to explain but may sacrifice performance. Striking the right balance—especially in regulated or high-stakes environments—is one of XAI’s toughest dilemmas.
2. Tooling and Integration Gaps
Many XAI tools exist, but integrating them into production systems can be technically demanding. Some platforms lack native support, and explanation techniques like SHAP can be resource-intensive. Teams may also face skill gaps and unclear best practices.
3. Organizational Resistance
Change does not come easy. Developers may view XAI as extra work, and leadership might not prioritize it—until a compliance issue or failure emerges. Gaining buy-in requires education, governance alignment, and proof of value.
4. Explanation Limitations
XAI tools offer approximations, not absolute truths. Methods like SHAP and LIME have their constraints, and poorly calibrated explanations can confuse more than clarify. Moreover, explanations expose issues—they don’t solve them.
Despite these challenges, explainability is becoming essential. Businesses that anticipate and address these hurdles early will be better equipped to deliver AI systems that are not only smart—but also transparent, trusted, and compliant.
Best Practices and Implementation Techniques
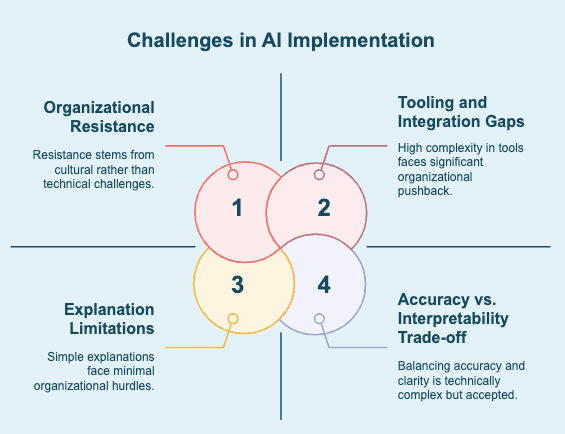
Successfully delivering Explainable AI (XAI) requires more than tools—it demands thoughtful integration, communication, and continuous evaluation.
Best Practices for Implementing XAI
- Embed Explainability Early
Plan for XAI from the start—during data prep, model selection, and validation—not as a bolt-on afterthought. Early integration ensures clarity and saves rework. - Match Methods to Use Case
No one-size-fits-all: SHAP and LIME work well for tabular data; Grad-CAM for images; attention maps for NLP. Choose methods based on whether you need local (individual decisions) or global (model-wide) insights. - Use Visuals to Simplify
Graphs, heatmaps, and bar charts make model behaviour digestible. For example, showing top feature contributions helps users instantly grasp why a decision was made. - Tailor for Non-Technical Users
Avoid jargon. Translate outputs into business terms. Use layered explanations—start with a summary, and offer deep dives if needed. - Monitor and Audit Continuously
Track explanation drifts like you monitor data drift. Review explanation logs, validate them regularly, and collect user feedback to refine how insights are communicated
To Deliver XAI, Businesses Can Use Techniques Such As
- Feature Attribution Tools
Leverage SHAP, LIME, and Integrated Gradients to explain which inputs influenced predictions and by how much. - Counterfactual Explanations
Show “what-if” scenarios—how slight input changes could alter outcomes, helping users understand thresholds and decision boundaries. - Model-Agnostic Explainers
Use tools that work across models (e.g., LIME) for flexibility, especially when dealing with complex black-box architectures. - Natural Language Summaries
Add plain-text explanations like: “Claim denied due to repeated entries and unusual amount.” These enhance user trust, especially in customer-facing systems. - Interactive Dashboards
Enable users to explore decisions, toggle inputs, and visualize impacts—boosting transparency and adoption across teams.
When implemented thoughtfully, XAI bridges the gap between technical complexity and human understanding—making your AI systems not only accurate, but also trusted, accountable, and usable.
Why Choose GeekyAnts as Your Explainable AI Development Services Provider?
At GeekyAnts, we blend cutting-edge AI capabilities with a strong focus on transparency and compliance. Our team of experts builds Explainable AI (XAI) solutions that are not only accurate but also auditable, user-friendly, and tailored to your industry’s needs—whether in healthcare, fintech, or enterprise tech.
We don’t integrate explainability tools as an afterthought—we design AI systems with interpretability at their core. Using frameworks like SHAP, LIME, and Grad-CAM, we deliver clarity behind every decision your model makes. From building internal dashboards to customer-facing explanation layers, we ensure every stakeholder understands and trusts your AI.
With over 17+ years of product engineering experience and deep expertise in AI, design systems, and scalable architecture, GeekyAnts helps you deploy AI that’s not only smart but also safe, transparent, and regulation-ready.
Ready to build AI systems your users can trust? Let’s get started.
Conclusion
As AI becomes integral to business operations, explainability has shifted from a nice-to-have to a necessity. Explainable AI (XAI) addresses the trust gap by making AI systems transparent, accountable, and aligned with business values.
Beyond regulatory compliance, XAI offers a strategic edge—organizations that can clearly explain their AI decisions are more likely to earn the trust of clients, regulators, and partners. It transforms AI from a black box into a tool for ethical, informed, and auditable decision-making.
Adopting XAI now positions your business as a responsible innovator. It enhances governance, accelerates adoption, and ensures your AI systems deliver insights that people can understand—and act on.
In short, explainability builds better AI—trusted, sustainable, and ready to scale.
Frequently Asked Questions (FAQs)
1. How does explainability in AI impact regulatory compliance?
Many regulations—including GDPR, HIPAA, and the EU AI Act—require that AI-driven decisions be explainable. XAI helps generate human-readable justifications, proving that models do not rely on protected attributes like race or gender. It ensures traceability, supports audits and reduces legal and ethical risks.
2. What are the main techniques for achieving explainable AI?
Common XAI techniques include:
- SHAP & LIME: Quantify how each input influenced the outcome.
- Feature Importance: Rank key factors driving predictions.
- Visual Tools: Heatmaps for vision, attention maps for text.
- Counterfactuals: Show how slight input changes alter outcomes.
- Surrogate Models: Use simpler models to explain complex ones.
These tools are model-agnostic, open-source, and widely supported by AI platforms.
3. How can businesses ensure their AI models are interpretable to non-technical users?
Design explanations for your audience. Use plain language summaries, clear visuals, and interactive dashboards. Avoid technical jargon. Offer layered insights—quick takeaways for executives, and detailed views for analysts. Always connect the AI’s logic to business outcomes or user impact.
4. Are there any downsides to implementing explainable AI?
Yes, a few. Some explanation methods are computationally heavy and may slow real-time systems. Explanations can be misinterpreted if oversimplified or taken as causal. Too much transparency may expose proprietary logic. And not all users will grasp technical outputs without training. Still, with the right tools and strategy, the benefits far outweigh the trade-offs.
Dive deep into our research and insights. In our articles and blogs, we explore topics on design, how it relates to development, and impact of various trends to businesses.