Table of Contents
How AI and Machine Learning are Strengthening Fraud Detection in Fintech
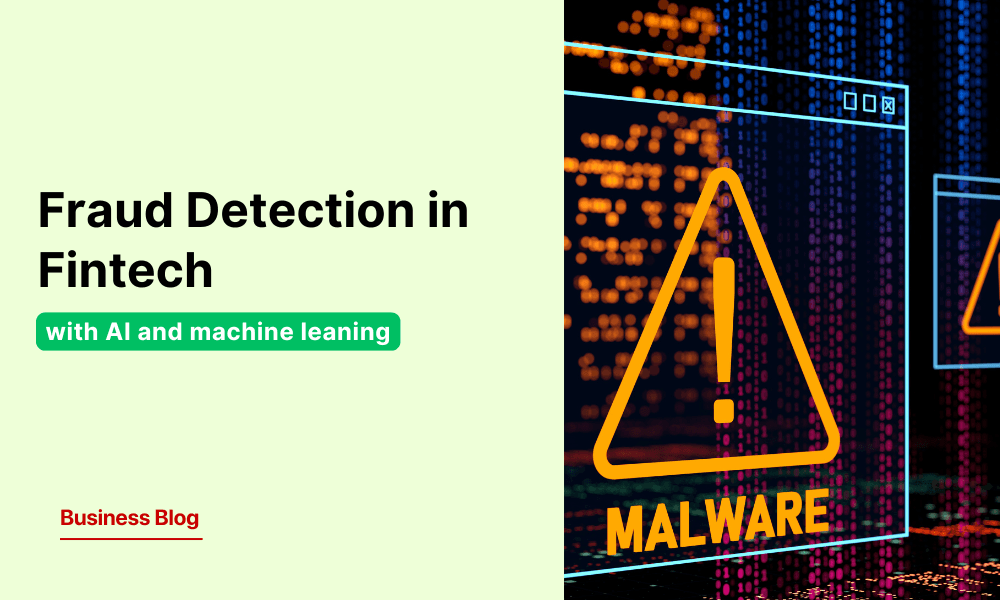
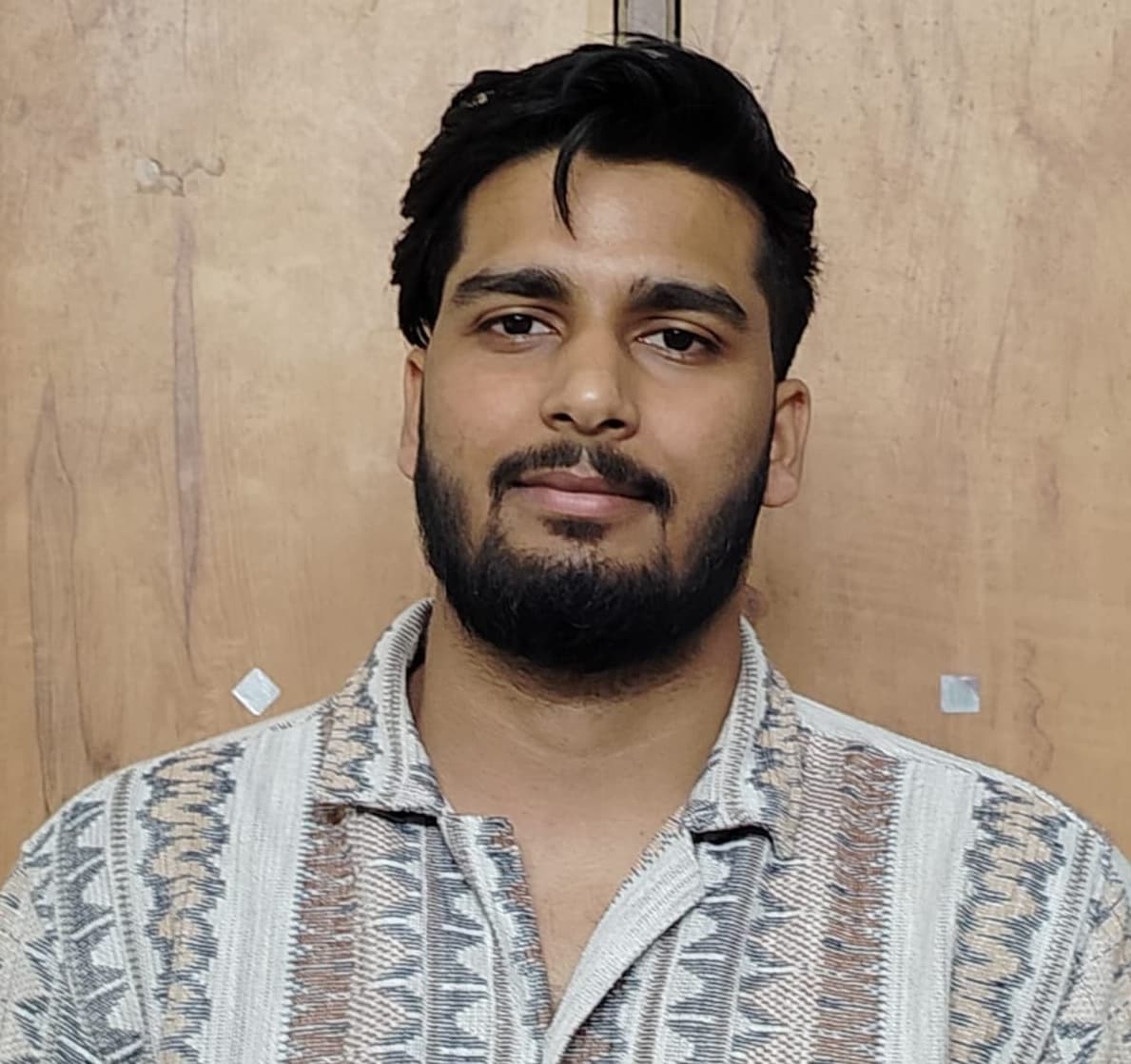
Book a call
In fintech, speed drives growth—but also creates openings for fraud. As real-time payments and digital banking scale, so do the risks.
In 2023, global losses from online payment fraud crossed $41 billion (Statista), exposing the limits of traditional fraud detection systems. Built on static rules and delayed reviews, they often miss evolving threats, flag legitimate users, and slow down transactions.
To stay ahead, fintechs are adopting AI and machine learning (ML)—technologies that detect anomalies in real time, adapt to new fraud patterns, and protect users without adding friction.
In this blog, we will explore how AI is strengthening fraud prevention across fintech—from replacing outdated systems to powering real-world, enterprise-scale defence.
Traditional vs AI-Powered Fraud Detection
Traditional fraud detection systems rely on static rules and manual review, such as flagging all transactions above a fixed threshold. While easy to implement, these systems are reactive, rigid, and prone to high false positives. They often miss complex fraud patterns that evolve quickly.
AI-powered systems, on the other hand, use real-time behavioral data and pattern recognition to detect subtle anomalies. They adapt with each transaction, identify fraud before it escalates, and reduce the need for manual intervention.
Rather than replacing human oversight, AI enhances it—offering faster detection, deeper insights, and greater precision.
Feature | Traditional Systems | AI-Powered Systems |
Detection Method | Rule-based, static | Adaptive, behavior-based |
Speed | Delayed detection | Real-time response |
False Positives | High | Significantly reduced |
Pattern Recognition | Known patterns only | Learns and flags unknown patterns |
Scalability | Limited by manual processes | Easily scales across millions of transactions |
How AI/ML Are Applied in Fintech Fraud Detection
Modern fintech platforms are deploying AI/ML across the entire fraud detection lifecycle. Key techniques include:
- Anomaly Detection: Identifies deviations from a user's normal behavior—like multiple logins from different geographies within minutes.
- Behavioural Biometrics: Uses indicators such as typing speed, mouse movement, and mobile tilt to distinguish between genuine users and bots or fraudsters (e.g., BioCatch).
- Predictive Modeling: Learns from historical fraud patterns to anticipate emerging fraud schemes—without relying on pre-defined rules.
- Natural Language Processing (NLP): Helps in KYC by parsing identity documents, understanding customer queries, and spotting intent anomalies.
- Graph Machine Learning: Detects fraud rings by mapping relationships across users, devices, accounts, and transactions (e.g., identifying shared devices or IPs used in multiple fraudulent cases).
These methods work together to deliver layered fraud defence, ensuring that signals are not evaluated in isolation, but in a rich context.
Real-World Implementations and Results
AI-driven fraud detection has moved beyond theory—it is actively shaping the operational backbone of leading financial platforms.
At PayPal, AI models scan over 1 billion transactions per day, identifying and intercepting fraudulent activity in milliseconds. This precision allows them to neutralize threats without disrupting legitimate transactions—a critical balance in a high-volume ecosystem.
JPMorgan Chase has integrated machine learning into its fraud alert systems, enabling smarter prioritization of threats. This shift has significantly lowered false positives and improved the efficiency of fraud investigation teams.
Meanwhile, in India, the National Payments Corporation (NPCI) is embedding AI across the UPI network to secure one of the fastest-growing digital payment ecosystems in the world. AI strengthens fraud prevention while supporting real-time transactions across millions of users.
Across regions and use cases, AI is proving its value-enhancing accuracy, reducing friction, and scaling protection without scaling human costs.
Business Benefits of AI-Driven Fraud Detection
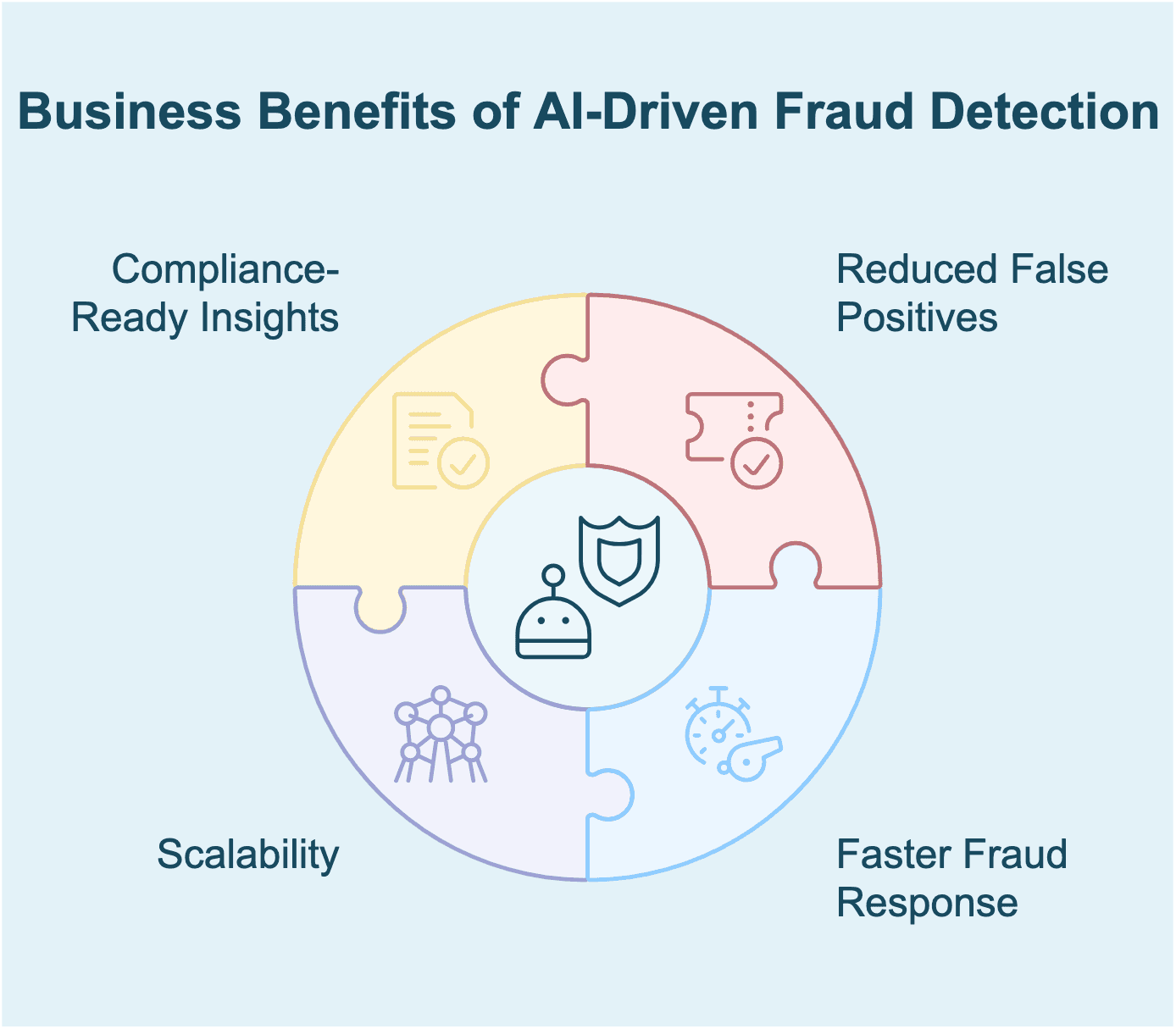
Beyond security, AI offers significant business and operational value:
- Reduced false positives: Fewer legitimate transactions are incorrectly blocked, improving customer experience.
- Faster fraud response: Instant alerts allow platforms to act before damage occurs.
- Scalability: AI models can evaluate millions of transactions in parallel—something manual systems cannot match.
- Compliance-ready insights: With built-in audit trails, AI helps fintechs meet regulatory reporting requirements more efficiently.
As fraud grows more complex, AI enables fintechs to protect at scale—without compromising growth or trust.
Challenges and the Road Ahead
While AI offers speed and scale, deploying it in fraud detection comes with critical challenges. Model bias—often stemming from incomplete or unbalanced datasets—can lead to unfair outcomes, disproportionately flagging certain user segments. Regulatory compliance adds pressure, with laws like GDPR and RBI guidelines demanding transparency in every automated decision.
The lack of explainability in traditional AI models—the so-called “black-box” problem—poses a major hurdle for risk and compliance teams. As a result, the shift toward Explainable AI (XAI) is accelerating, making fraud decisions more auditable and accountable.
Looking forward, technologies like federated learning, edge AI, and blockchain-based fraud registries are emerging as solutions to improve privacy, decentralization, and performance. Tackling these challenges will define how fintech builds resilient, trustworthy fraud prevention systems in the years ahead.
Conclusion
Fraud prevention is evolving into a strategic differentiator—shaping how fintechs scale, earn trust, and meet compliance demands. Success now depends on intelligent systems that adapt in real time, detect subtle anomalies, and respond before damage occurs.
AI and machine learning bring the capability to anticipate threats, reduce false positives, and streamline risk operations at enterprise scale. These systems don’t sit on the periphery—they’re embedded into the fabric of modern financial infrastructure.
Talk to our AI experts at GeekyAnts to deploy secure, real-time fraud prevention solutions purpose-built for high-growth fintech platforms.
Dive deep into our research and insights. In our articles and blogs, we explore topics on design, how it relates to development, and impact of various trends to businesses.