Table of Contents
AI in Healthcare: From Innovation to Integration
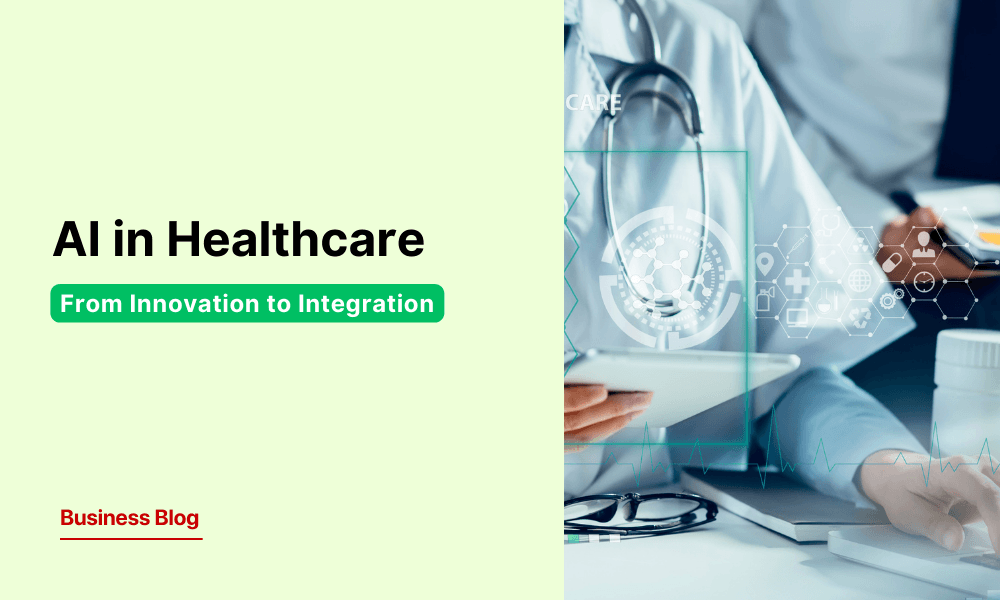
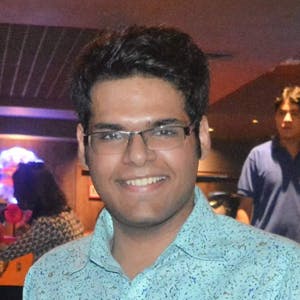
Book a call
What if AI could detect diseases before symptoms appear, personalize treatments in real-time, and automate complex diagnostics? This is not a distant vision—it is happening now. AI is no longer an auxiliary tool in healthcare but a core driver of medical innovation, enhancing everything from radiology and diagnostics to patient monitoring and personalized care.
The shift is undeniable—in 2023 alone, the FDA approved over 200 AI-powered medical devices, a sharp rise from just six approvals in 2015. This surge reflects the healthcare industry’s growing reliance on AI to improve efficiency, reduce diagnostic errors, and enable faster clinical decision-making.
In this blog, we explore how AI is transforming healthcare, its role in medical devices, personalized care, diagnostics, ethical challenges, and the future of AI-powered patient care.
AI-Powered Medical Devices: Enhancing Accuracy and Efficiency
AI-driven medical devices are redefining diagnostics, patient monitoring, and treatment delivery, moving beyond automation to proactive, real-time intelligence. From AI-assisted radiology scans that detect anomalies faster to ICU ventilators that adjust airflow dynamically, these innovations optimize clinical workflows and improve patient outcomes.
The integration of AI into wearable and implantable devices enables continuous health monitoring, early disease detection, and personalized treatment recommendations. However, model reliability, data security, and bias in AI training remain critical challenges as these technologies become more autonomous. Ensuring regulatory compliance, interoperability with electronic health records, and ethical AI deployment will shape the future of AI-powered healthcare.
Personalized Healthcare: Scaling Patient Engagement Through AI
Healthcare is moving beyond traditional doctor-dependent models, with AI enabling scalable, data-driven personalization. By analyzing patient history, biomarkers, and real-time vitals, AI tailors health recommendations that support preventive care, chronic disease management, and fitness optimization.
AI-powered platforms detect patterns, predict health risks, and issue early alerts, allowing patients to take proactive measures before symptoms escalate. Apple’s AI-driven cardiac rhythm detection is a prime example, already helping individuals identify atrial fibrillation early, potentially preventing life-threatening events.
However, ensuring medical accuracy, eliminating bias, and maintaining ethical integrity remain critical challenges. AI should function as a decision-support tool, empowering both patients and healthcare professionals while adhering to rigorous validation and ethical standards.
The Rise of AI in Radiology and Diagnostics
Radiology was among the first areas to experience an AI-driven transformation, largely due to the high volume of imaging data and repetitive analysis involved in diagnostics. AI-powered X-rays, MRIs, and ultrasound scans are now enhancing the precision of radiology assessments, detecting anomalies that human specialists might overlook.
This shift is reflected in regulatory trends—radiology-based AI applications make up a significant portion of FDA-approved AI-driven medical devices. AI’s ability to analyze large imaging datasets, reduce diagnostic errors, and streamline reporting has made it an essential tool in modern radiology departments.
However, AI in radiology is not without risks. False positives, algorithmic bias, and data integrity challenges must be addressed through rigorous validation models. AI is an enabler, not a replacement—radiologists will continue to play a crucial role in interpreting AI-generated findings and ensuring clinical accuracy.
AI vs. Generative AI in Healthcare: Understanding the Difference
AI and Generative AI (Gen AI) are often used interchangeably, but in healthcare, they serve distinct roles. While AI powers predictive analytics, automation, and data-driven diagnostics, Gen AI specializes in natural language processing, chatbot interactions, and automated documentation.
Aspect | AI in Healthcare | Generative AI (Gen AI) in |
Primary Function | Predictive analytics, automation, diagnostics | Natural language processing, content generation |
Applications | Medical imaging analysis, disease prediction, treatment recommendations | Chatbots, AI-driven triage, medical transcription |
Clinical Use Cases | Radiology, patient monitoring, drug discovery | Virtual assistants, administrative support |
Regulatory Status | Widely adopted, with FDA-approved medical devices | No FDA-approved devices for clinical decision-making |
Key Challenges | Data privacy, bias, reliability | Risks of hallucinations, misinformation, and lack of validation |
Role in Healthcare | Assists in medical decision-making | Supports documentation and patient interactions |
While Gen AI has improved efficiency in patient engagement and administrative workflows, it is not yet clinically validated for direct medical decision-making due to concerns about accuracy, bias, and regulatory approval. As healthcare AI continues to evolve, maintaining a balance between automation and human oversight will be crucial for clinical integrity.
Ethical and Security Challenges in AI-Driven Healthcare
As AI becomes more embedded in healthcare, it raises critical ethical and security challenges, particularly around patient privacy, data security, and algorithmic bias. AI systems process vast amounts of sensitive health data, making HIPAA compliance, encryption, and secure storage essential to prevent breaches and unauthorized access.
One of the most pressing concerns is bias in AI models. If trained on incomplete or skewed datasets, AI can produce inaccurate recommendations, disproportionately affecting certain demographics and widening healthcare disparities. Continuous audits, diverse dataset training, and bias detection protocols are necessary to ensure fairness and reliability in AI-driven insights.
Another challenge is AI hallucinations, where systems generate false or misleading medical suggestions. Without strict validation frameworks and human-in-the-loop oversight, these inaccuracies could compromise patient safety. AI is a powerful enabler, but its outputs must be clinically validated, cross-checked by professionals, and implemented with caution to uphold trust and medical accuracy.
Future of AI in Healthcare: What Lies Ahead?
AI has already reshaped diagnostics, medical devices, and patient engagement, yet its most transformative potential remains untapped. The key question now is: When will healthcare experience its 'ChatGPT moment'—a breakthrough that fully integrates AI into clinical workflows and decision-making? The answer depends on scaling AI responsibly, securing regulatory approvals, and ensuring seamless interoperability across global healthcare systems.
In the coming years, AI is expected to:
- Revolutionize diagnostics by serving as a virtual second opinion, enhancing accuracy and reducing misdiagnosis.
- Advance precision medicine through real-time biomarker analysis, enabling personalized treatments tailored to individual genetic profiles.
- Improve AI-driven preventive care, detecting early warning signs of diseases before symptoms manifest, reducing hospitalizations.
- Shape regulatory advancements, ensuring AI adoption aligns with ethical standards, clinical validation, and patient safety.
The true test of AI’s impact in healthcare will lie in its ability to deliver reliable, unbiased, and clinically validated insights at scale. As AI, data analytics, and regulatory frameworks evolve, the healthcare industry will move closer to an era where AI is not just an assistant but an indispensable pillar of modern medicine.
Conclusion
AI is redefining healthcare, shifting from assistive technology to a critical pillar of diagnostics, treatment planning, and patient management. Its future hinges on scalable deployment, regulatory alignment, and precision-driven automation while maintaining clinical integrity and ethical AI governance.
Success will depend on rigorous validation, bias mitigation, and seamless integration into clinical workflows. Organizations that adopt AI with a data-driven, compliance-first approach will lead the next phase of intelligent, outcome-focused healthcare.
Dive deep into our research and insights. In our articles and blogs, we explore topics on design, how it relates to development, and impact of various trends to businesses.