Table of Contents
Agentic AI and Its Core Components: Empowering Machines to Think—But at What Cost?
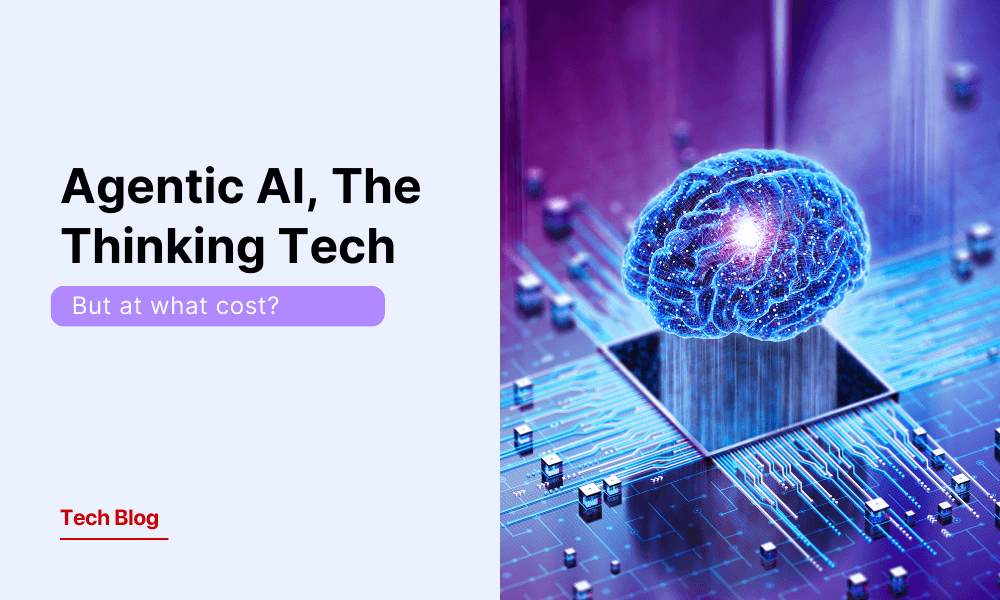
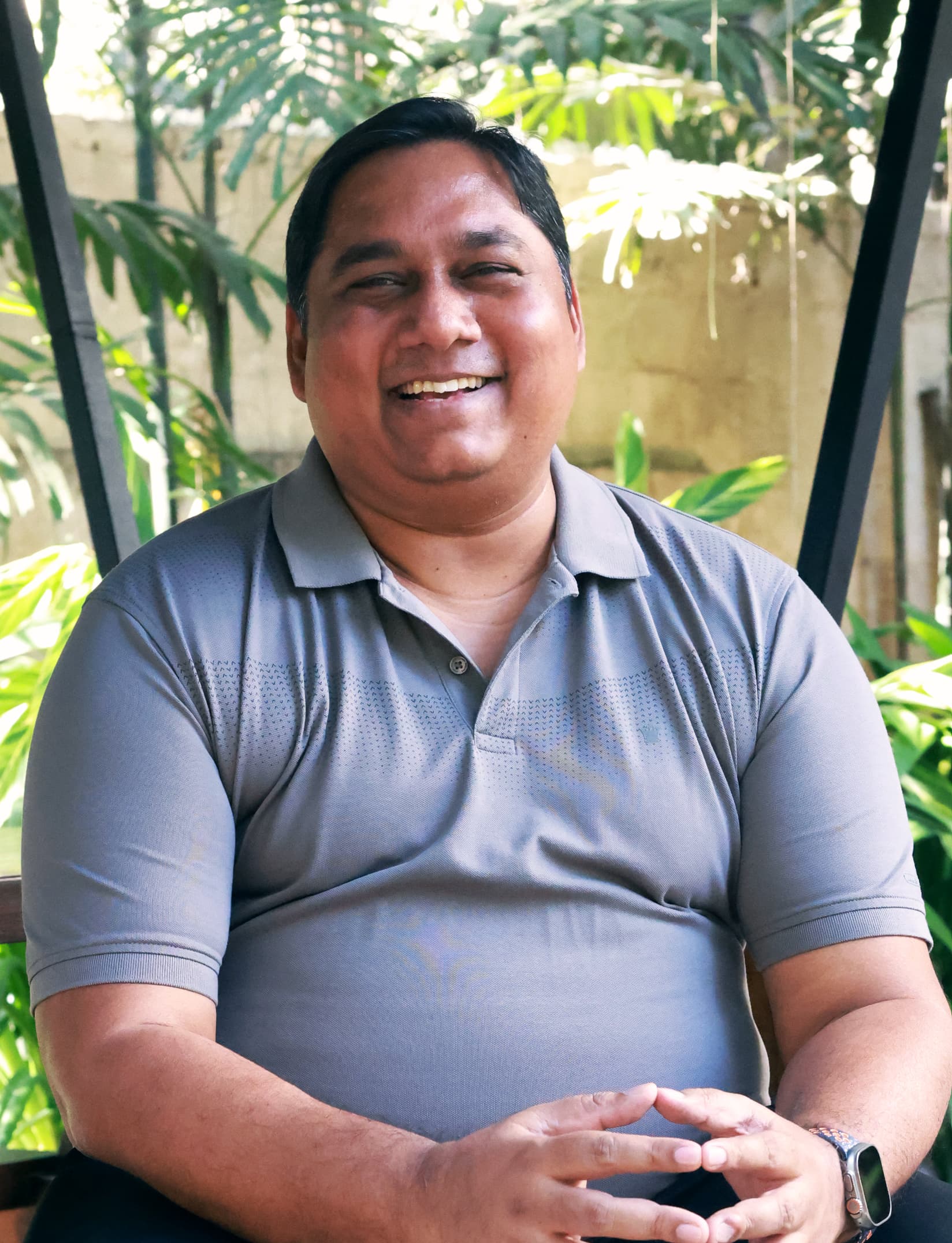
Book a call
Agentic AI systems are transforming the technological landscape by operating independently, making decisions, and taking action to achieve specific goals. Unlike traditional software that follows rigid, predefined steps, Agentic AI is designed to perceive its environment, learn from experiences, adapt to new situations, and act autonomously. This capability has significant implications for industries such as autonomous vehicles, healthcare diagnostics, and financial trading, where real-time decisions can greatly influence outcomes.
At GeekyAnts, we are actively driving innovation in this field. Our focus is on building advanced AI solutions that enable businesses and developers to unlock the potential of Agentic AI. We aim to create systems that can function independently, adapt to changing circumstances, and continuously improve.
Key Components of Agentic AI
Agentic AI is structured into several interconnected layers, each contributing to its intelligence, adaptability, and reliability.
I. Perception Layer
The Perception Layer is responsible for gathering, organizing, and interpreting data to provide the foundation for decision-making.
- Data Acquisition
This step involves collecting information from various sources, such as sensors, online data feeds, or user inputs. Examples include cameras for autonomous vehicles or APIs for financial applications. - Data Processing
Once data is collected, it is processed to ensure it is useful. This involves removing irrelevant information, standardizing formats, and highlighting critical features. - Environmental Awareness
Contextual understanding allows the AI to interpret data more effectively. For example, it can detect anomalies like unexpected traffic patterns or unusual market fluctuations.
II. Cognition Layer
The Cognition Layer focuses on setting goals, making decisions, and storing knowledge for future use.
- Goal Formulation
This involves defining what the AI system is expected to accomplish. Goals may include short-term objectives, such as avoiding obstacles, and long-term targets, such as navigating complex routes. - Decision-Making Models
The system analyzes data and selects the best course of action. Techniques like reinforcement learning, planning algorithms, and rule-based systems are often used to guide decision-making. - Knowledge Representation
This step involves organizing learned information into formats that can be easily retrieved and utilized. The AI may use knowledge graphs or databases to store facts, relationships, and rules.
III. Action Layer
The Action Layer enables the AI system to execute decisions in the real or virtual world.
- Actuation Mechanisms
This step involves translating decisions into tangible actions. In robotics, this could mean moving robotic arms, while in software, it might involve triggering specific functions. - Execution Monitoring
The system monitors its actions to ensure they are carried out as planned. If errors occur, the AI can adjust its approach or issue alerts. - Adaptive Behavior
Learning from past actions allows the AI to improve over time. By analyzing outcomes, the system refines its responses to handle similar situations more effectively.
IV. Collaboration Layer
The Collaboration Layer emphasizes interaction between AI systems, humans, and ethical frameworks.
- Multi-Agent Communication
In complex environments, multiple AI agents must work together. This communication ensures coordination, as seen in distributed systems or drone swarms. - Human-AI Interaction
This step involves providing users with insights into what the AI is doing and why. Transparency helps build trust and allows users to offer feedback or make corrections. - Ethical Compliance
Ethics are integral to AI design. Mechanisms are implemented to ensure fairness, prevent biased outcomes, and comply with guidelines for responsible use.
V. Feedback Loop
The Feedback Loop ensures the system evolves by learning from new data and experiences.
- Continuous Learning
The AI updates its models and strategies as it encounters new information. This ensures the system remains relevant and effective. - Performance Evaluation
The system regularly assesses its performance, identifying areas for improvement and maintaining reliability. - Error Handling
If unexpected issues arise, the AI employs predefined strategies to address them or alerts humans for intervention. This ensures resilience and reliability.
Summing Up
Agentic AI transcends the boundaries of traditional systems by enabling machines to perceive, reason, act, and adapt without constant human intervention. Its intricate framework, composed of Perception, Cognition, Action, Collaboration, and Feedback layers, empowers it to operate intelligently in dynamic and challenging environments. This transformative capability marks a pivotal step forward in the evolution of artificial intelligence.
At GeekyAnts, we are proud to be at the forefront of this technological revolution. By embedding the foundational principles of Agentic AI into our solutions, we strive to create systems that are not only intelligent and adaptive but also reliable and ethically sound. We believe that the power of Agentic AI will redefine industries, spark innovation, and positively impact lives worldwide. Together, we can shape a future driven by intelligent, purposeful technology.
Dive deep into our research and insights. In our articles and blogs, we explore topics on design, how it relates to development, and impact of various trends to businesses.